Exploring New Directions on Robustness in Machine Learning
On November 16, IDEAL hosted a workshop focused on new directions on robustness in machine learning as part of the fall 2021 special quarter.
Machine learning systems are widely deployed to facilitate decision-making. They’re used for many tasks — ranging from image and speech recognition, to medical diagnostics and electronic health record data mining, to securities trading and financial fraud detection — making it vital for the systems to be reliable and secure against adversarial corruptions.
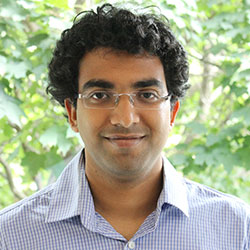
Robustness describes how much a system or algorithm can withstand and tolerate data errors, contamination, and misspecification during training, modeling, and testing phases.
Vijayaraghavan and Jason Hartline, professor of computer science in the McCormick School of Engineering, are co-directors of the Institute for Data, Econometrics, Algorithms, and Learning (IDEAL). Launched in 2019 by a team of interdisciplinary investigators at Northwestern, Toyota Technological Institute at Chicago, and the University of Chicago, IDEAL studies the theoretical foundations related to high dimensional data analysis, data science in strategic environments, and machine learning and optimization.
On November 16, IDEAL hosted a workshop focused on new directions on robustness in machine learning as part of the fall 2021 special quarter organized by Vijayaraghavan; Chao Gao, assistant professor of statistics at the University of Chicago; and Yu Cheng, assistant professor of mathematics at the University of Illinois at Chicago.
“During the fall special quarter, we studied some of the foundational questions about when and how we can design methods for machine learning and high-dimensional estimation that are robust and reliable,” said Vijayaraghavan.
Workshop speakers explored novel notions of robustness and the different challenges that arise in designing reliable and secure machine learning algorithms. Discussion topics included test-time robustness, adversarial perturbations, and distribution shifts.
Kamalika Chaudhuri, associate professor of computer science and engineering at the University of California San Diego, discussed the robustness of training algorithms to small, imperceptible perturbations to legitimate test inputs, or adversarial examples, that cause machine learning classifiers to misclassify.
Pranjal Awasthi, research scientist at Google, studies theoretical machine learning with a particular focus on designing robust algorithms for unsupervised learning.
Sébastien Bubeck, senior principal research manager for machine learning foundations at Microsoft Research, discussed joint research he conducted with Mark Sellke, a fourth-year graduate student in mathematics at Stanford University, that illustrates why robustness necessitates large neural networks
Aleksander Mądry, Cadence Design Systems Professor of Computing at MIT, presented a direct training data-to-output model that is a versatile framework for analyzing machine learning predictions.
Gautam Kamath, assistant professor of computer science at the University of Waterloo, specializes in robust statistics and data privacy. He surveyed different problems and results on differential privacy arising in the context of various statistical estimation settings.
Jinshuo Dong, IDEAL postdoctoral fellow, also helped organize the November event.
IDEAL’s next special quarter, “High Dimensional Data Analysis,” starts in spring 2022 and will include graduate courses, workshops, and reading groups. The spring quarter is being organized by Konstantin Makarychev, professor of computer science at Northwestern Engineering, and Yury Makarychev, professor of computer science at the Toyota Technological Institute at Chicago.
IDEAL is led by co-principal investigators from the three participating institutions. The Northwestern team also includes:
- Randall Berry, John A. Dever Chair of Electrical and Computer Engineering
- Dongning Guo, professor of electrical and computer engineering and (by courtesy) computer science
- Samir Khuller, Peter and Adrienne Barris Chair of Computer Science
- Zhaoran Wang, assistant professor of industrial engineering and management sciences and (by courtesy) computer science
- Eric Auerbach, assistant professor of economics at the Kellogg School of Management
- Ivan Canay, HSBC Research Professor of Economics at Kellogg
- Joel Horowitz, Charles E. and Emma H. Morrison Professor of Economics at Kellogg
IDEAL is a Harnessing the Data Revolution (HDR) Transdisciplinary Research in Principles of Data Science (TRIPODS) institute supported by the National Science Foundation under award CCF 1934931.