Examining the Intersection of Machine Learning and Mathematical Logic
IDEAL presents an introduction to machine learning and logic
The intersection of machine learning and mathematical logic — spanning computer science, pure mathematics, and statistics — has catalyzed recent advances in artificial intelligence and deep learning toward solving complex problems with predictive models.
On February 28, the Institute for Data, Econometrics, Algorithms, and Learning (IDEAL) hosted a workshop introducing researchers to problems involving the fields of machine learning and logic.
The event was organized as part of the IDEAL Winter/Spring 2023 Special Program on Machine Learning and Logic by James Freitag, associate professor of mathematics, statistics, and computer science at the University of Illinois at Chicago (UIC); and Lev Reyzin, professor of mathematics, statistics, and computer science and IDEAL site director at UIC.
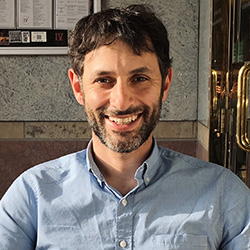
Northwestern’s IDEAL site director Aravindan Vijayaraghavan, associate professor of computer science and (by courtesy) industrial engineering and management sciences at Northwestern Engineering, welcomed attendees to the workshop held in Mudd Hall, hosted by Northwestern Computer Science. He noted the expertise across disciplines represented by IDEAL investigators, including computer science, economics, electrical engineering, law, mathematics, operations research, and statistics.
This special program is the first of IDEAL Phase 2, which aims to accelerate transformative advances in the theoretical foundations of data science through research and education programs on machine learning and optimization; high-dimensional data analysis and inference; and emerging topics including reliability, interpretability, privacy, and fairness. IDEAL is a multidisciplinary collaboration across Northwestern, Google Research, the Illinois Institute of Technology, the Toyota Technological Institute at Chicago, UIC, and the University of Chicago.
Program
The workshop began with overviews of machine learning and logic, followed by a research talk at their intersection.
Reyzin outlined foundational notions of computational learning theory, including probably approximately correct (PAC) learning, online learning, and statistical queries. He also related these learning models to different notions of dimension, including Vapnik–Chervonenkis (VC) dimension, Littlestone, and statistical query (SQ).
Freitag discussed Littlestone dimension and its origins in model theory. He also connected trees and half-graphs via a Ramsey-type theorem argument related to Littlestone dimension and its dual.
Maryanthe Malliaris, professor of mathematics at the University of Chicago, presented joint work with Shay Moran, professor of mathematics at Technion – Israel Institute of Technology, titled “The Unstable Formula Theorem Revisited via Algorithms.” The work proves that Littlestone classes characterize learnability in a new statistical model and sets the stage for an approximation theorem characterizing Littlestone classes in terms of a range of learning models.
Upcoming events
On April 10-14, the five participating universities of IDEAL will host the “Workshop on Machine Learning, Interpretability, and Logic,” organized by Reyzin, Shai Ben-David, professor of computer science at the University of Waterloo, and Gyorgy Turan, professor of mathematics, statistics, and computer science at UIC.