EECS & HABits Lab Students Shibo Zhang, Rawan Alharb, & William Stogin Achieve Best Paper Award at 2016 BODYNETS
Their research focuses on using wrist-worn sensors to detect & count fine grained activity (feeding gestures) using a passive sensing data analytic framework.
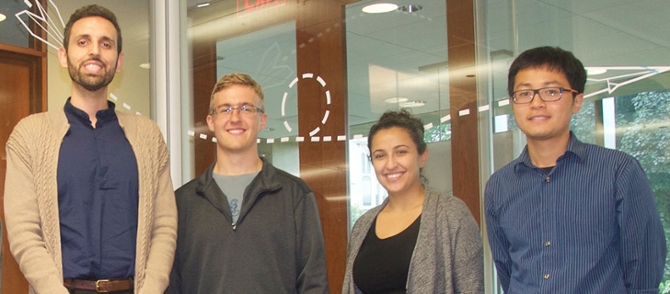
The Health Aware Bits (HABits) Lab, including EECS PhD Students Shibo Zhang, Rawan Alharbi, and Undergraduate Student William Stogin (CS 18'), recently received the Best Paper Award at BODYNETS 2016: 11th International Conference on Body Area Networks (BodyNETS), held Dec. 15-16, 2016 in Turin Italy.
Their paper, titled, "Food Watch: Detecting and Characterizing Eating Episodes through Feeding Gestures", also featured co-authors Prof. Mohammad Pourhomayoun (Computer Science at California State University, Los Angeles), Prof. Angela F. Pfammatter (Feinberg School of Medicine, Northwestern University), Prof. Bonnie Spring (Weinberg College of Arts and Sciences, Northwestern University), and Prof. Nabil Alshurafa whom advises all three students.
"The paper focused on using wrist-worn sensors to detect and count fine grained activity (feeding gestures) using a passive sensing data analytic framework. Existing obesity and behavioral research focuses on burdensome dietary self-report, and by reliably counting and identifying feeding gestures, behaviorists can now passively (without user burden) detect and characterize eating episodes and design interventions that predict, prevent, and ultimately treat problematic eating behaviors", said Prof. Alshurafa.
Abstract Excerpt: Obesity, caused primarily by overeating, is a preventable chronic disease yielding staggering healthcare costs. And while existing passive sensors combined with machine learning algorithms are not yet able to reliably passively detect caloric overeating, they are beginning to reliably detect how and when people eat. With the ubiquitous nature of wrist-worn sensors, existing literature has focused on detecting eating episodes in hopes of aiding users in dietary recall. Our objective is to use wearable sensors, not only to aid in dietary recall, but to objectively identify problematic eating episodes. We analyze the effect of time-series segmentation and sensing configurations on the accuracy of detecting and characterizing feeding gestures.
The Health Aware Bits (HABits) Lab designs, builds and analyzes end-to-end mHealth systems, while focusing on processing its data to help answer health-related questions. The lab focuses on signal processing and machine learning techniques to process time-series data generated from passive sensors. To advance existing sensing techniques, a part of the lab focuses on the design of embedded systems.
BODYNETS 2016 aims to provide a world-leading and unique opportunity for bringing together researchers and practitioners from diverse disciplines to plan, analyze, design, build, deploy and experiment with/on Body Area Networks (BANs).
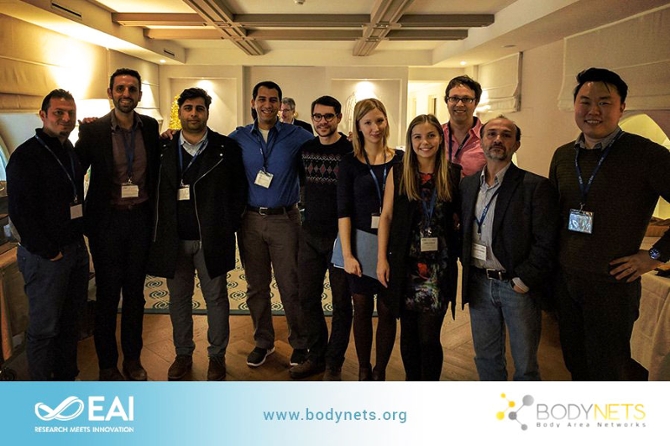