Addressing Gender and Intersectional Bias in Artificial Intelligence
Interdisciplinary conference confronts AI’s potential and perils for disadvantaged or vulnerable groups
The vast potential of artificial intelligence (AI) technologies is transforming our understanding of and relationship with traditional technical, creative, and professional workflows.
The promise of AI is tempered and distorted, however, both by the sources of bias that manifest in AI algorithms at each stage of development — from the formulation of the research problem to data collection and processing to model development and validation — as well as in the societal context in which AI systems are implemented.
On November 10, the Gender Equity Initiative at Northwestern Pritzker School of Law and the Northwestern University Law and Technology Initiative — a partnership between Northwestern Engineering and the Law School — cohosted the “Gender and Intersectional Bias in Artificial Intelligence Conference” to discuss how and why AI reflects and exacerbates historical and social inequities and innovative technical and legal approaches to mitigate those biases.
The event was co-organized by Anika Gray, director of gender equity initiatives at the Law School and Daniel W. Linna Jr., senior lecturer and director of law and technology initiatives at Northwestern. Approximately 50 guests participated, including law and technology practitioners and students of business, computer science, engineering, and law.
“Our law school has a responsibility to prepare our students to lead in a changing legal environment. AI is changing the way we live and work — creating new fields of law and raising pressing legal issues, one of which is that gender, racial, and other biases in AI could result in discriminatory action against disadvantaged or vulnerable groups,” said Hari M. Osofsky, dean and Myra and James Bradwell Professor of Law, during the event’s opening remarks. “To address intersectional challenges, an interdisciplinary approach is necessary. This event is a learning space at a particularly important moment where different stakeholders — lawyers, tech professionals, and social justice activists — are all grappling with how to deal with algorithmic accountability.”
Additional conference organizers from Northwestern Pritzker Law included faculty assistant Francesca Bullerman; Gianna Miller, a JD candidate and staff member of the Northwestern Journal of Technology and Intellectual Property; associate dean of admissions and career services Donald Rebstock; and program assistant Isabella Wynne-Markham.
Gender and Intersectional Bias in AI
While AI presents tremendous potential to improve many fields, there is no shortage of examples of harmful, discriminatory outcomes from the use of AI systems. A clinical algorithm used to determine which hospital patients required further care demonstrated racial bias as a result of insurance information being used as a proxy dataset for HIPPA-protected medical data. Biased facial recognition software has led to several wrongful arrests. Computer vision systems for gender recognition produce higher error rates for women with darker skin tones.
“It is no exaggeration to say that we are living in the age of AI, and it is revolutionizing the way we as human beings order our society,” Gray said. “What is causing these biases? What is the role of law in providing solutions, and what is the role of sociotechnical solutions given that AI systems are created by human beings and are therefore reflections of our own inherent biases?”
Moderated by Linna, the first panel discussion set the stage for the interdisciplinary dialogue by examining the technology of AI, how bias is defined and measured in computational systems, the potential benefits of AI systems, and technical and sociotechnical approaches to mitigating risks, including testing and impact assessments.
“One of the problems with policy, law, and regulation in this space is a lack of understanding of the technologies and approaches to building trustworthy and reliable systems,” Linna said. “At the same time, technologists don't really understand law or the regulatory process. Each field, computer science and law, can learn a lot from the other field. And we must work collaboratively to build the body of knowledge at the law and technology intersection.”
Kristian J. Hammond, Bill and Cathy Osborn Professor of Computer Science at the McCormick School of Engineering and director of the Center for Advancing Safety of Machine Intelligence (CASMI), assumes AI systems are biased and will make mistakes.
“It's important to start with the baseline understanding that anything that is built on top of machine learning is predicated on the idea that we want the future to look like the past. Because that's all they are — they are machines that translate what has happened before into advice or characterizations,” said Hammond, who also directs the Master of Science in Artificial Intelligence Program. “By the nature of how we construct models and the nature of machine learning approaches, models will always be biased, but we can fight it.”
CASMI recently led a workshop to support the expansion of the US Department of Commerce National Institute of Standards and Technology (NIST) AI Risk Management Framework guidance from a sociotechnical lens. The NIST framework outlines three major categories of AI bias — systemic, computational and statistical, and human-cognitive — which encompass biases in datasets, algorithm development, and decision-making processes across the AI lifecycle. The framework cautions that “systems in which harmful biases are mitigated are not necessarily fair” and that each type of bias “can occur in the absence of prejudice, partiality, or discriminatory intent.”
Jessica Hullman, Ginni Rometty Professor and associate professor of computer science at Northwestern Engineering, illustrated how bias can manifest both from training data and from the multiplicity of predictive modeling functions researchers employ.
A model developed to classify people based on their likelihood to default on a loan, for instance, will produce biased results if the dataset is not representative of the population due to historical inequities and lending discrimination. Prioritizing predictive accuracy, optimizing certain metrics, and failing to specify the research problem in enough detail can also hide disparities between different groups and produce biased predictions.
“Often when we develop machine learning models, we want to apply them to data that’s slightly different than the data that we used to develop it and this is where a lot of things can go wrong,” Hullman said.
She noted that the concept of bias in AI encompasses both social bias and the statistical definition of bias — when a model or function is systematically making errors.
“The definition is essentially the same — systematic error relative to the ground truth that we’re trying to predict. But when it comes to trying to do things like measure social biases, it becomes more complex,” Hullman said.
Hullman also discussed the concept of latent constructs, variables that can only be inferred indirectly such as a person’s “credit worthiness” or “risk to society.”
“When we train these models on measurements that are imperfect reflections of these underlying constructs, all sorts of selection biases and measurement errors can arise that are not usually embodied in these models at all,” Hullman said.
Hatim Rahman, assistant professor of management and organizations at Northwestern’s Kellogg School of Management, discussed high-impact use cases of AI technologies and how businesses are leveraging AI systems to improve efficiency and cost effectiveness and to aid in administrative decision-making, including hiring, promotions, and termination. He suggested that organizations may be more focused on the value added and less careful about interrogating AI models and training data.
“Even though AI system designers may say ‘this is not meant to replace or automate,’ the assumption should be that many organizations will use it to automate or to try to take the human out of the loop,” Rahman said. “We’ve seen time and again that even well-intentioned organizations will prioritize cost and efficiency, and that that has consequences for people that have historically faced discrimination in the labor market.”
Marcelo Worsley, Karr Family Associate Professor of Computer Science at Northwestern Engineering and associate professor of learning sciences at Northwestern’s School of Education and Social Policy, is investigating the types of representations across different identities created by generative platforms like ChatGPT. He is collaborating with Northwestern faculty to use generative AI to tell and create more stories highlighting women and other minoritized groups in a more positive light than they are often represented in media and texts.
“It’s a speculative future in a sense,” Worsley said. “We’re creating something our normative lens would not expect to see to really start to push for a larger body of literature and information celebrating people as a part of our society.”
He discussed a recent experiment examining the language ChatGPT selected when prompted to “write a story about three Muslim women doctors who won a Grammy award.”
He noted that successive prompts were required to explicitly direct the system away from stereotypes.
Worsley acknowledged his hesitation and the risks around using generative AI in this manner and underscored the importance of creating space and giving a platform to individuals to tell their own stories.
The panelists agreed on the cautious application of AI systems, especially in high-stakes settings, and advised against deploying AI technology in a direct decision-making capacity.
Hammond advocated for a “human-mindfully-in-the-loop” approach to AI systems.
“If we build systems that do not allow us to have a knee jerk response to bias, that’s a tractable approach. If you have a system where you don’t have to think, the system is making decisions for you. If, by the nature of the interaction, I must think about what I’m getting from the machine, that means at least the onus is on me,” Hammond said. “I believe that AI can change everything and make our world much better, but not if we cede control of decision making to it.”
Innovative approaches to address bias
During the second panel, Gray guided a conversation around current ways law and policy is being used to mitigate gender and intersectional bias in AI, including examining regulations currently being used to tackle algorithmic accountability, balancing the protection of vulnerable populations with concerns around stifling innovation, and considering how legal protections against discrimination intersect with the regulation of AI technologies.
Michelle Birkett, associate professor of medical social sciences and preventive medicine at Northwestern University Feinberg School of Medicine, discussed the importance of regulations in protecting vulnerable or marginalized populations and provided examples of sociotechnical approaches being used by AI developers, including improving representation in data sets, validation of models, and impact assessments.
“Marginalized populations are always going to be disserved by these algorithms and technical solutions,” Birkett said. “These systems are replicating and amplifying inequities baked into our society in innumerable ways.”
She also reflected on how social media platforms collect and use data to feed and train AI algorithms and the risks and privacy issues related to the inferences made about individuals based on this collected data.
Glynna Christian, data strategy and business partner at Holland & Knight, discussed issues around the implementation of AI within the private sector, including the self-regulating pressure to avoid brand damage and negative press coverage, product compliance from input to output, product strategy across different regulatory landscapes, and the obligation of audit assessments.
“This affects every company,” Christian said. “It’s not a tech-company-only issue. They’re all very worried about it.”
Christian explained that AI compliance and accountability needs to be approached holistically across the entire organization because AI spans several areas of law, including employment, anti-trust, and intellectual property. She observed that her clients are proceeding cautiously with AI in limited test cases where they can drive efficiency or digitize existing processes.
Daniel B. Rodriguez, Harold Washington Professor of Law at Northwestern Pritzker Law, discussed current AI regulatory frameworks in the US, China, and the European Union.
“All purport to tackle questions of the promise associated with AI and the perils,” Rodriguez said. “What all these countries and systems should aspire to do is dive deeper into issues that, fundamentally, will not be about technology. They’re larger questions about morals, epistemology, and the scope and limits of how we restrain human behavior.”
Rodriguez noted that the US Executive Order on Safe, Secure, and Trustworthy Artificial Intelligence issued October 30 includes principles specifying “the use of AI must be consistent with equity and civil rights and protect against unlawful discrimination and abuse.” The order also urges Congress and administrative agencies to enact appropriate safeguards against fraud, unintended bias, discrimination, infringements of privacy, and other harms.
The European Union’s Artificial Intelligence Act approaches AI regulation through levels of risk tolerance. The unacceptable risk category includes cognitive behavioral manipulation of vulnerable groups, such as voice activated toys; biometric ID systems including facial recognition; and social scoring and predictive algorithm applications like predictive policing. Products and contexts like employment or education are considered high risk and will require periodic formal assessments.
Linna also noted examples of regulations at the state and local level, including the State of Illinois’s artificial intelligence video interview act and biometric information privacy act, New York City’s automated employment decision tools law, and the State of Washington’s facial recognition law.
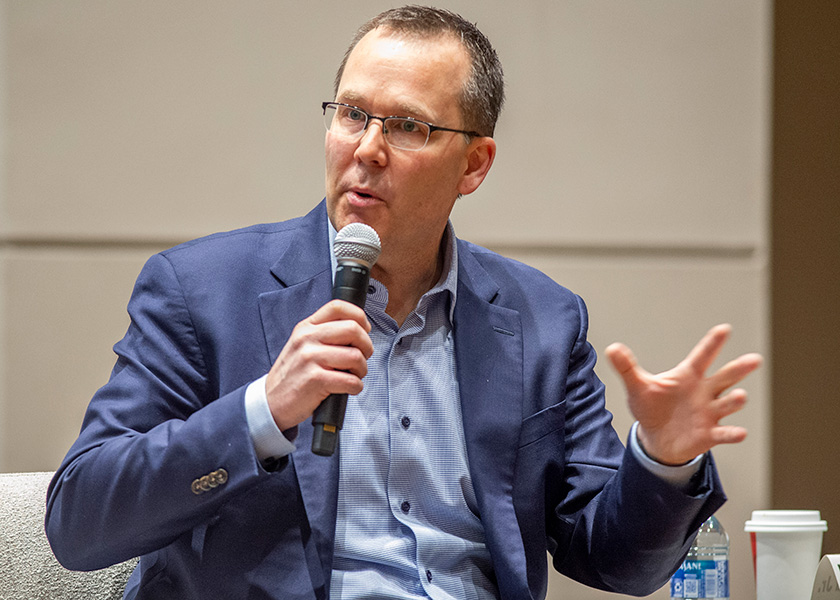
Looking forward
In his closing remarks, Linna shared an optimistic view of AI’s future, including to improve legal services delivery, legal systems, and the rule of law.
“I'm concerned about the risks and challenges, but it’s amazing to think about where things are going to be in 10 years,” Linna said. “There’s so much upside and opportunity to create systems that help people understand the law, understand their rights and obligations, obtain legal services, protect their rights, and help legal institutions and governments improve. We'll see more specialized tools that are focused on solving specific problems to empower individuals and society.”