Faculty DirectoryJessica Hullman
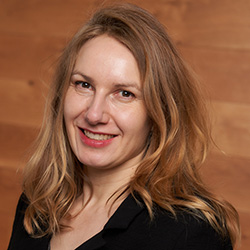
Ginni Rometty Professor of Computer Science
Contact
2233 Tech DriveMudd Room 3521
Evanston, IL 60208-3109
Email Jessica Hullman
Website
Departments
Education
Tableau Software Postdoctoral Fellowship, Computer Science Division, University of California Berkeley, Berkeley, CA, 2015
Master of Science in Information (Information Analysis and Retrieval), The University of Michigan School of Information, Ann Arbor, MI, 2008
Bachelor of Arts, Comparative Studies. The Ohio State University, Columbus OH, 2003
Research Interests
My interests lie in challenges and limitations that arise when people theorize and draw inductive inferences from data. My research on uncertainty representation and interactive data analysis explores how to best align data-driven interfaces and summaries with human reasoning capabilities, how to understand the role of interactive analysis across different stages of a statistical workflow, how to evaluate data interfaces as well as the experiments researchers use to identify differences between them, and how to develop tools that support reasoning under uncertainty in domains like strategic games or privacy. I approach many of these problems by drawing on formal models of rational inference as a basis for comparison and proposing solutions.
Selected Publications
Zhang, D., Chatzimparmpas, A., Kamali, N., and Hullman, J. Evaluating the Utility of Conformal Prediction Sets for AI-Advised Image Labeling. ACM CHI 2024.
Zhang, D., Hartline, J., and Hullman, J. Designing Shared Information Displays for Agents of Varying Strategic Sophistication. ACM CSCW 2024.
Wu, Y., Guo, Z., Mamakos, M., Hartline, J., and Hullman, J. The Rational Agent Benchmark for Data Visualization. IEEE TVCG 2023.
Kale, A., Guo, Z., Qiao, E., Heer, J., and Hullman, J. EVM: Incorporating Model Checking into Exploratory Visual Analysis. IEEE TVCG 2023.
Subramonyam, H., and Hullman, J. Are We Closing the Loop Yet? Gaps in the Generalizability of VIS4ML Research. IEEE TVCG 2023.
Gelman, A., Hullman, J., Kennedy, L. Causal Quartets: Different Ways to Attain the Same Average Treatment Effect. American Statistician, 2023.