Funding New Research to Operationalize Safety in Artificial Intelligence
Center for Advancing Safety of Machine Intelligence Awards $2.2 Million
The Center for Advancing Safety of Machine Intelligence (CASMI) at Northwestern University, a collaboration with the UL Research Institutes' Digital Safety Research Institute (DSRI), is providing $2.2 million in funding for eight new projects across seven institutions.
The projects will help advance CASMI’s mission to operationalize a robust safety science in artificial intelligence (AI), in part by broadening its network of researchers, who will work to improve outcomes.
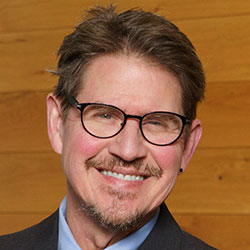
The projects were awarded in November 2022, following an open call for research proposals. Each project was eligible for up to $275,000 in funding for two years.
The principal investigators represent the following institutions: University of Minnesota, Northwestern University, University of Amsterdam, Carnegie Mellon University, University of Wisconsin-Madison, Purdue University, and Northeastern University.
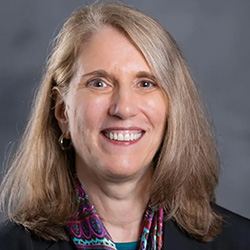
Principal investigators are researching various methods to quantify AI that is safe, equitable, and beneficial. Projects range from investigating safe and compassionate machine learning (ML) recommendations for people with mental illnesses to expressing human situations and contexts to machines.
This is the second group of projects CASMI has funded since its launch in April 2022. The initial group of projects has already produced promising results. Previous research has investigated the lack of reliability of algorithmic personality tests used in hiring. Last year, researchers also developed a framework to improve stress tests for autonomous vehicles. Another project identified data gaps in road safety by comparing rural and urban areas. CASMI researchers have also developed a Human Impact Scorecard to assess and to demonstrate an AI system’s impact on human well-being.
These new projects will address some of the critical research gaps and opportunities identified in the CASMI Research Roadmap. This includes studying data and ML algorithms to understand how the systems are designed to interact with people. Creating these building blocks is essential to establish a safety culture in AI.
Anticipating AI Impact in a Diverse Society: Developing a Scenario-Based, Diversity-Sensitive Method to Evaluate the Societal Impact of AI-Systems and Regulations
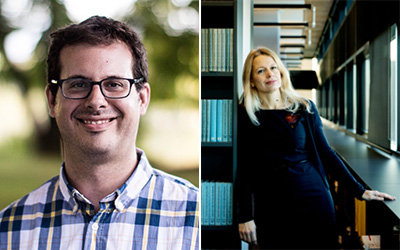
Nicholas Diakopoulos is collaborating with co-investigator Natali Helberger to develop a method to anticipate the impacts of new AI technologies by engaging diverse sets of stakeholders in scenario writing activities, or prospections, to envision the impacts of new AI technology in society.
Diakopoulos is an associate professor of communication studies in Northwestern’s School of Communication and (by courtesy) associate professor of computer science in Northwestern Engineering. Helberger is a distinguished university professor of law and digital technology at the University of Amsterdam, director of the AI, Media & Democracy Lab, and member of the board of directors for the Institute for Information Law (IViR).
Effective as orienting devices in decision-making processes, the goal of prospections is not to predict the future, but to perceive potential futures in the present and develop forward-looking evaluation frameworks. The team aims to develop a methodology to anticipate the impacts of new AI technologies and present a nuanced picture of future AI safety issues through diverse perspectives.
Co-designing Patient-Facing Machine Learning for Prenatal Stress Reduction
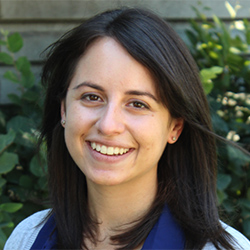
A cross-disciplinary collaboration between researchers in human-computer interaction, ML, and healthcare, the project aims to evaluate how algorithms can support pregnant people managing prenatal stress through three components of a next-day stress prediction model; namely the prediction, the explanation, and a recommendation to use a just-in-time stress management exercise.
Human-AI Tools for Expressing Human Situations and Contexts to Machines
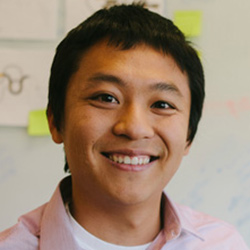
To bridge this gap and address significant concerns around safety, privacy, and inequitable access to AI-supported experiences, Haoqi Zhang, an associate professor of computer science at Northwestern Engineering, aims to advance new programming environments and tools that support designers in the construction of machine representations using available context features.
Zhang directs the Design, Technology, and Research (DTR) program and is a codirector of the Delta Lab.
Safe and Compassionate Machine Learning Recommendations for People with Mental Illnesses
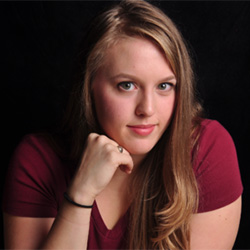
Chancellor’s team will design and evaluate one participant-centered machine learning (ML) intervention to alleviate algorithmic harms on social networks and build a system that makes safer and more compassionate recommendations for people in distress.
Chancellor is a former CS + X postdoctoral fellow in computer science at Northwestern Engineering, co-advised by Darren Gergle, John G. Searle Professor of Communication Studies in Northwestern’s School of Communication; and Sara Owsley Sood, Chookaszian Family Teaching Professor and associate chair for undergraduate education at the McCormick School of Engineering.
Dark Patterns in AI-Enabled Consumer Experiences
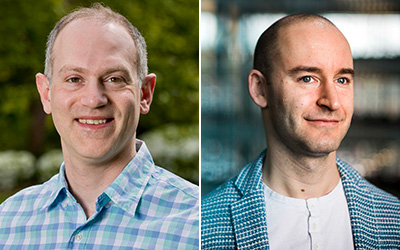
The team will investigate applications of AI in consumer electronics and third-party software to identify new classes of dark patterns and construct ground-truth datasets of dark pattern prevalence. Through user studies, Choffnes and Wilson also aim to better understand user perceptions about AI dark patterns and their potential to cause harm.
Supporting Effective AI-Augmented Decision-Making in Social Contexts
Holstein is working with co-investigators Haiyi Zhu, Steven Wu, Alex Chouldechova and PhD students Luke Guerdan and Anna Kawakami. The team's goal is to develop an understanding of how expert decision-makers work with AI-based decision support to inform social decisions in real-world contexts, and to develop new methods that support effective decision-making in these settings.
Understanding and Reducing Safety Risks of Learning with Large Pre-Trained Models
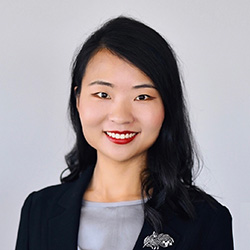
Sharon Yixuan Li, an assistant professor of computer sciences at the University of Wisconsin-Madison, aims to understand how pre-trained data models can exacerbate safety concerns and to mitigate the safety risks of transfer learning with large, pre-trained data models.
Li proposes a novel evaluation framework to comprehensively understand how inequity and out-of-distribution risks are propagated through the transfer learning process. She will then apply this framework to build new learning algorithms that enhance safety and de-risk the potential negative impacts when transferring knowledge from pre-trained models.
Diagnosing, Understanding, and Fixing Data Biases for Trusted Data Science
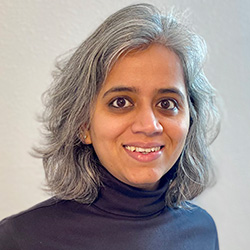
Pradhan will investigate how to diagnose bias in ML pipelines and evaluate and integrate the impact of data quality. By decoupling data-based applications from the mechanics of managing data quality, Pradhan aims to help practitioners more easily detect and mitigate biases stemming from data throughout their workflows.
Projects
The project investigators join CASMI’s existing research team, including Kristian Hammond, CASMI director and Bill and Cathy Osborn Professor of Computer Science at Northwestern Engineering; Michael Cafarella (MIT); Leilani H. Gilpin (University of California, Santa Cruz); Francisco Iacobelli (Northeastern Illinois University and Northwestern University); Ryan Jenkins (California Polytechnic State University); Julia Stoyanovich (New York University); and Jacob Thebault-Spieker (University of Wisconsin-Madison).