Faculty DirectoryFerdinando Mussa-Ivaldi
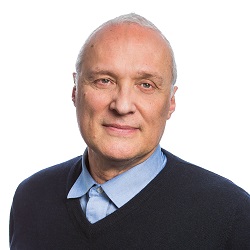
Professor of Physiology
Professor of Physical Medicine & Rehabilitation
Professor of Biomedical Engineering
Contact
(Physiology) Ward 5-009(RIC): Room 1304
312-238-1230Email Ferdinando Mussa-Ivaldi
Website
Robotics at the Rehabilitation Institute of Chicago
Other RIC research initiatives
Departments
Affiliations
Research Interests
Bi-Directional Brain-Machine Interfaces, Body Machine Interface for Controlling Assistive Devices, Computational primitives for sensory-motor learning, Motor adaptation to changes in arm dynamics, The Representation of Time in the Sensory-Motor System, Understanding Force Control
When young children learn to write a signature, their brains generate motor commands that are different from the commands needed to do the same thing as adults. And yet, we do not need to relearn handwriting as our bones and muscles change. Our sensory-motor is equipped with a powerful machinery that modifies the previously acquired motor programs so as to compensate for changes in the body and in the surrounding environment. The activities of our research group are directed at understanding the biological and computational mechanisms underlying this remarkable ability to learn and adapt. At the same time, we wish to take advantage of this knowledge for designing new procedures and technologies that facilitate the recovery of motor functions lost to stroke and other injuries.
Bi-Directional Brain-Machine Interfaces
We are carried out a collaborative study for the development of brain-machine interfaces that may establish a bi-directional communication between the nervous system and external devices. This requires decoding neural activities for extracting motor intentions and creating patterns of stimulation that encode physical variables associated with the mechanical state of the controlled device. A long-term goal of this study is to facilitate the neural control through the control of the neural plasticity mechanisms that are normally allowing for motor learning.
Body Machine Interface for Controlling Assistive Devices
Stroke and spinal cord injury survivors may experience severe difficulties in maneuvering and steering their wheelchairs. The combination of a person's limited mobility and an interface that requires a precise and inflexible manipulation is a source of problems. This highlights the need for the development of control interfaces that are tailored to the specific residual motor skills of the user. Motion tracking technology offers a convenient and flexible way of capturing the motions of a disabled user with limited mobility, as sensors can be placed virtually anywhere on the body and measure very small motions. We are investigating and developing new methods for controlling assistive devices (e.g. powered wheelchairs) using wearable sensors. Controlling the external device (wheelchair) is practiced in a virtual reality environment, which allows the device's interface to gradually change so as to fit with the patient's evolving skills. At the end of this process, we plan to apply the evolved interface to the actual device (wheelchair or robot) and test the efficacy of learning in the real-life context.
Computational primitives for sensory-motor learning
A number of electrophysiological studies have revealed a modular organization within the structure of the spinal cord. When a small electrical stimulation is delivered to a site in the lumbar spinal cord, a group of muscle is activated. The result of this activation is a field of forces that tend to drive the ipsilateral hindlimb toward a stable posture. Most remarkably, the simultaneous stimulation of multiple sites leads to a vectorial summation of the force fields generated by each site. These studies have suggested that the brain may generate complex motor behaviors by adding the force fields produced by multiple modules in the spinal cord. We are developing a theory of motor control based of the superposition of these force fields, which we consider as "motor primitives" in analogy with the language primitives used to generate unlimited sentences out of a finite vocabulary of words.
Motor adaptation to changes in arm dynamics
To generate even the simplest movements of the arm, the brain must solve a complex problem of dynamics. The relation between the forces generated by the muscles of the arm and the ensuing movement is expressed by a system of complex nonlinear differential equations. A number of studies have suggested that the brain maintains an internal representation of this dynamical relation, not in the form of a mathematical expression but in the form of a transformation from desired movement to corresponding command. This type of representation has been called an "internal model". We are investigating the properties of internal models by observing how subjects interact with a robotic manipulator that applies a preprogrammed force field to their hand. This force fields constitutes a change in the dynamics that the subject's motor system must represent in order to move the hand as desired. The current experimental evidence indicate that the subjects have an accurate representation of the dependence of the force upon the state of motion of the limb. However, there is a surprising inability to represent correctly a perturbation that depends explicitly upon time. We wish to pursue this analysis to understand what are the mechanisms and the limitations of our ability to adapt to changes in limb dynamics.
The Representation of Time in the Sensory-Motor System
Advanced technology provide us with truly immersive virtual environments, including teleoperated robotic devices. In order to control movements from a distance, the human sensorimotor system has to overcome the effects of delay. Currently, little is known about the mechanisms that underlie haptic estimation in delayed environments. The objective of this study is to determine the effect of sensory and environmental delays on perception of surfaces stiffness. The results of the experiment indicate a systematic dependence of the estimated stiffness upon the delay between position and force. Understanding the neural processing of temporal information has implications that go far beyond technological application. How is time represented (if at all) in the nervous system? How does the brain recognize that different sensory events – for example, the sound and the force impulse generated by the impact of the hand with an object – are to be considered as simultaneous despite different neural delays? Do we process simultaneity as an equivalence relation? Answering these questions is critical to understand sensory-motor processing.
Understanding Force Control
When manipulating objects, we must control our hand motion as well as the interaction forces that arise from contact with the environment. At the level of musculoskeletal biomechanics, motions and forces are coupled by intrinsic limb impedance. However, it has yet to be established whether at the neural level the control of motion and force are coupled or independent. The objective of this study is to understand how the nervous system simultaneously controls hand movements and interaction forces during active haptic discrimination. We have found results suggesting the existence of independent neural controllers for arm motion and interaction forces. This evidence is offered by transcranial magnetic stimulation of posterior parietal cortex resulting in the differential disruption of the control of motion but not of force.
Read a more detailed and extended list of research projects that are ongoing in the Robotics Laboratory of the Rehabilitation Institute of Chicago.
Selected Publications
Farshchian, A., A. Sciutti, A. Pressman, I. Nisky and F. A. Mussa-Ivaldi (2018). "Energy exchanges at contact events guide sensorimotor integration across intermodal delays." eLife 7: e32587.
Thorp, E. B., K. P. Kording and F. A. Mussa-Ivaldi (2017). "Using noise to shape motor learning." Journal of Neurophysiology 117: 728-737.
Pierella, C., F. Abdollahi, E. Thorp, A. Farshchiansadegh, J. Pedersen, I. Seáñez-González, F. A. Mussa-Ivaldi and M. Casadio (2017). "Learning new movements after paralysis: Results from a home-based study." Scientific Reports 7(1): Article n.4779.
Abdollahi, F., A. Farshchiansadegh, C. Pierella, I. Seáñez-González, E. Thorp, M.-H. Lee, R. Ranganathan, J. Pedersen, D. Chen, E. Roth, M. Casadio and F. A. Mussa Ivaldi (2017). "Body-Machine Interface Enables People With Cervical Spinal Cord Injury to Control Devices With Available Body Movements: Proof of Concept." Neurorehabilitation and Neural Repair 3(1): 487-493.
Farshchiansadegh, A., A. Melendez-Calderon, R. Ranganathan, T. D. Murphey and F. A. Mussa-Ivaldi (2016). "Sensory Agreement Guides Kinetic Energy Optimization of Arm Movements during Object Manipulation." PLoS Comput Biol 12(4): e1004861.
Thorp, E. B., F. Abdollahi, D. Chen, A. Farshchiansadegh, M.-H. Lee, J. Pedersen, C. Pierella, E. J. Roth, I. Seanez-Gonzalez and F. A. Mussa-Ivaldi (2015). "Upper Body-Based Power Wheelchair Control Interface for Individuals with Tetraplegia." IEEE Transactions on Neural Systems and Rehabilitation Engineering 24(2): 249 - 260.
Pierella, C., F. Abdollahi, A. Farshchiansadegh, J. Pedersen, E. B. Thorp, F. A. Mussa-Ivaldi and M. Casadio (2015). "Remapping residual coordination for controlling assistive devices and recovering motor functions." Neuropsychologia 79, Part B: 364-376.
Casadio, M., A. Pressman and F. A. Mussa-Ivaldi (2015). "Learning to push and learning to move: the adaptive control of contact forces." Frontiers in computational neuroscience 9:118.
Wang, X., M. Casadio, K. A. Weber, F. A. Mussa-Ivaldi and T. Parrish (2014). "White Matter Microstructure Changes Induced by Motor Skill Learning utilizing a Body Machine Interface." Neuroimage 88: 32-40.
Ranganathan, R., A. Adewuyi and F. A. Mussa-Ivaldi (2013). "Learning to be Lazy: Exploiting redundancy in a novel task to minimize movement-related effort." Journal of Neuroscience 33(7): 2754-2760.
Vato, A., M. Semprini, E. Maggiolini, F. D. Szymanski, L. Fadiga, S. Panzeri and F. A. Mussa-Ivaldi (2012). "Shaping the dynamics of a bidirectional neural interface." PLoS Computational Biology 8(7): e1002578.
Shadmehr, R. and F. A. Mussa-Ivaldi (2012). Biological Learning and Control. How the Brain Builds Representations, Predicts Events and Makes Decisions. Cambridge, MA, MIT Press.
Danziger, Z. and F. A. Mussa-Ivaldi (2012). "The influence of visual motion on motor learning." Journal of Neuroscience 32(29): 9859 –9869.
Pressman, A., A. Karniel and F. A. Mussa-Ivaldi (2011). "How Soft is That Pillow? The Perceptual Localization of the Hand and the Haptic Assessment of Contact Rigidity." Journal of Neuroscience 31: 6595-6604.
Mussa-Ivaldi, F. A., S. T. Alford, M. Chiappalone, L. Fadiga, A. Karniel, M. Kositsky, E. Maggiolini, S. Panzeri, V. Sanguineti, M. Semprini and A. Vato (2010). "New perspectives on the dialogue between brains and machines." Frontiers in Neuroscience 4(1): 44-52.
Casadio, M., A. Pressman, A. Fishbach, Z. Danziger, S. Acosta, D. Chen, H.-Y. Tseng and F. A. Mussa-Ivaldi (2010). "Functional reorganization of upper-body movement after spinal cord injury." Experimental Brain Research 207: 233-247.
Danziger, Z. C., A. Fishbach and F. A. Mussa-Ivaldi (2009). "Learning Algorithms for Human-Machine Interfaces." IEEE Transactions on on Biomedical Engineering 56: 1502-1511.
Chib, V. S., M. A. Krutky, K. M. Lynch and F. A. Mussa-Ivaldi (2009). "The Separate Neural Control of Hand Movements and Contact Forces " Journal of Neuroscience 29: 3939 - 3947.
Kositsky, M., A. Karniel, S. Alford, K. M. Fleming and F. A. Mussa-Ivaldi (2003). "Dynamical Dimension of a Hybrid Neuro-Robotic System." IEEE Transactions on Neural Systems and Rehabilitation 11: 155-159.
Scheidt, R. A., J. B. Dingwell and F. A. Mussa-Ivaldi (2001). "Learning to move amid uncertainty." Journal of Neurophysiology 86: 971-985.
Reger, B. D., K. M. Fleming, V. Sanguineti, S. Alford and F. A. Mussa-Ivaldi (2000). "Connecting brains to robots: an artificial body for studying the computational properties of neural tissue." Artificial Life 6: 307-324.
Mussa-Ivaldi, F. A. and E. Bizzi (2000). "Motor learning through the combination of primitives." Philosophical Transactions of the Royal Society. London B 355: 1755-1769.
Mussa-Ivaldi, F. A. (1999). "Modular features of motor control and learning." Current Opinion in Neurobiology 9: 713-717.
Conditt, M. A., F. Gandolfo and F. A. Mussa-Ivaldi (1997). "The motor system does not learn the dynamics of the arm by rote memorization of past experience." Journal of Neurophysiology 78(1): 554-560.
Gandolfo, F., F. A. Mussa-Ivaldi and E. Bizzi (1996). "Motor learning by field approximation." Proceedings of the National Academy of Sciences of the United States of America 93(9): 3843-3846.
Shadmehr, R. and F. A. Mussa-Ivaldi (1994). "Adaptive Representation of Dynamics During Learning of a Motor Task." Journal of Neuroscience 14: 3208-3224.
Mussa-Ivaldi, F. A. and N. Hogan (1991). "Integrable Solutions of Kinematic Redundancy Via Impedance Control." The International Journal of Robotics Research 10: 481-491.
Mussa-Ivaldi, F. A., P. Morasso and R. Zaccaria (1988). "Kinematic Networks. A Distributed Model for Representing and Regularizing Motor Redundancy." Biological Cybernetics 60: 1-16.
Mussa-Ivaldi, F. A. (1988). "Do Neurons in the Motor Cortex Encode Movement Direction? An Alternative Hypothesis." Neuroscience Letters 91: 106-111.
Mussa-Ivaldi, F. A., N. Hogan and E. Bizzi (1985). "Neural, Mechanical and Geometric Factors Subserving Arm Posture in Humans." Journal of Neuroscience 5: 2732-2743.
Morasso, P. and F. A. Mussa-Ivaldi (1982). "Trajectory Formation and Handwriting: A Computational Model." Biological Cybernetics 45: 131-142.