CASMI, TRAILS and FAS Collaborating with Federal Standards Body to Assess AI Impacts and Risks
Participants focused on evaluation, metrics, and measurement of AI systems through a sociotechnical lens
As the United Kingdom hosts the world's first global artificial intelligence (AI) safety summit, scholars from academia, industry, and government are also brainstorming how to improve standards in the United States to reduce AI risks and assess whether the technologies perform as intended.
The Northwestern Center for Advancing Safety of Machine Intelligence (CASMI) designed and led a workshop to support the expansion of the National Institute of Standards and Technology (NIST) AI Risk Management Framework (AI RMF) guidance from a sociotechnical lens. CASMI co-hosted the workshop with TRAILS – the NIST-National Science Foundation (NSF) Institute for Trustworthy AI in Law & Society – and the Federation of American Scientists (FAS) on Oct. 16-17 at The George Washington University (GW) in Washington, D.C., to discuss the sociotechnical methods and processes that are necessary to develop a testbed, or a controlled environment to measure and validate AI systems.
“I really do believe that AI can be phenomenal in what it can do for us as a species,” said Kristian Hammond, Bill and Cathy Osborn professor of computer science and director of CASMI, “but the goal is to make it safe while doing so.”
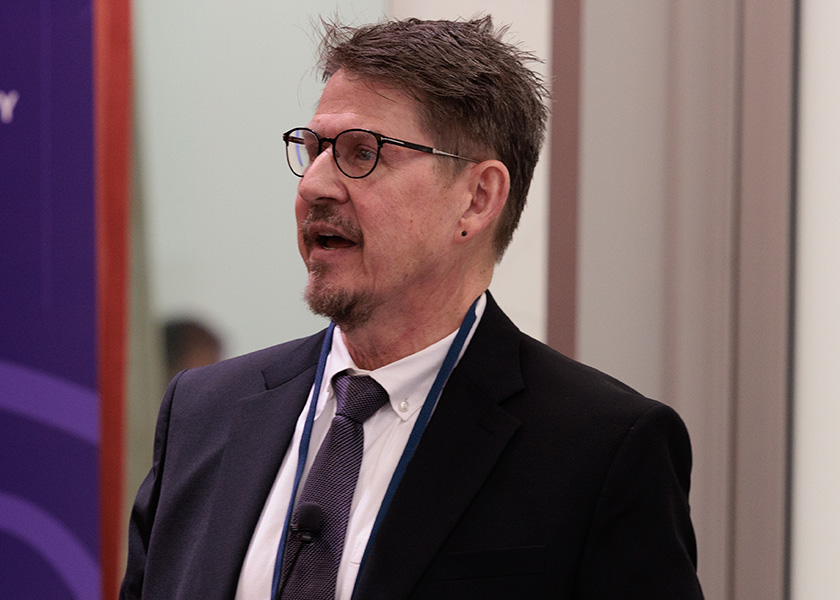
Abigail Jacobs, assistant professor of information and of complex systems at the University of Michigan, collaborated with TRAILS and FAS to help CASMI organize the workshop, entitled “Operationalizing the Measure Function of the NIST AI Risk Management Framework.”
“We were thrilled to co-host this workshop with CASMI and FAS on this crucial topic. The mission of TRAILS is to make the AI design process more participatory, and convenings like this, which involve representatives from government, industry, academia, and civil society organizations, are crucial to carrying out this mission,” said TRAILS GW lead and co-PI, David A. Broniatowski.
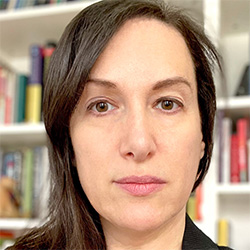
The workshop included presentations, question-and-answer sessions, a fireside chat, and breakout sessions. One of the speakers was Cathy O’Neil, a pioneer in algorithmic auditing and author of the bestselling book, Weapons of Math Destruction.
“The NIST AI RMF is a really good start at measuring the harms of AI,” said O’Neil, CEO of O'Neil Risk Consulting & Algorithmic Auditing (ORCAA). “People have complained to me that it’s not specific enough, but it can’t be. Every bureaucracy that we interact with as humans has been replaced by an algorithm in the last 30 years. The idea of having a single setup where we decide what fairness, safety, and risk management look like for every bureaucracy is silly.”
Developing a Sociotechnical Testbed
The NIST AI Risk Management Framework is a voluntary document that was released in January to guide organizations with methods to increase the trustworthiness of AI systems. One of its core functions is to measure AI risks using interdisciplinary and diverse perspectives.
“Identifying risks is the name of the game,” said Schwartz, who serves as principal investigator on bias in AI for NIST’s Trustworthy and Responsible AI program. “Once risks are identified – which is no trivial matter – they can be mapped, measured, and managed.”
Measuring risks also involves evaluating AI systems for trustworthy characteristics, continuously tracking risks, and assessing whether measurement tactics are working.
While the NIST AI RMF does not explicitly mention a testbed, Schwartz gave a presentation at the workshop detailing NIST’s upcoming approaches to evaluation through the creation of a sociotechnical, multi-purpose test environment called ARIA (which stands for Assessing Risks and Impacts of AI). It would focus on how risks and impacts arise from the ways different AI actors engage and interact with AI technology, and require new metrics to measure trustworthiness and impact.
“With a human subject test environment, we can observe interactions in a privacy-protected and controlled manner,” Schwartz said. “We can evaluate whether the technology performs safely, measure the probability of the occurrence of risks, and identify whether the risks outweigh the benefit.”
To estimate risks of machine learning systems, Schwartz said existing test approaches can be used together, such as A/B testing, which compares two variations within a model to see which one performs better; and red teaming, which involves independent parties testing systems to find vulnerabilities.
Focus on Addressing Embedded Harms in Systems
CASMI researchers are working to address the issues that AI is currently causing. This includes reducing bias in systems, identifying interfaces that may contribute to users making harmful decisions, and developing tailored systems for underserved communities.
Chowdhury’s presentation at the workshop focused on sociotechnical methods for measuring the impact of AI. She said that while the technical community’s approach tends to be narrowly focused on mathematical estimations, the social sciences have a rich history of creating flexible measurements of social concepts.
In her talk, Chowdhury drew from her experience leading industry teams to discuss the difficulty of translating user feedback into actionable insights for model developers, leading to both frustrated end users and product owners. Measurement of qualitative concepts – a common practice in quantitative social science – can build a bridge between concept and application. Historically, we have seen this take the shape of living indices for government transparency, corruption, social capital, wellbeing and more.
Chowdhury emphasized the importance of gathering constant user feedback about AI harms and risks, developing systematic measurement tools, and constantly updating those tools as AI models evolve.
“How can we create a way of doing this that keeps up with the pace at which models are refreshed, reused, and updated for different purposes?” she asked.
‘We Are Flying Blind’ — Designing a Cockpit for AI
Mathematician and data scientist Cathy O’Neil likes to use a metaphor to explain why we need to measure AI harms. Imagine you go into a plane and realize there is no cockpit or pilot.
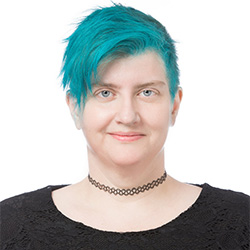
O’Neil’s company, ORCAA, has been auditing algorithms in context since 2016. Her team uses a framework, called explainable fairness, to define and measure when an algorithm is complying with an anti-discrimination law.
“It decides what kind of worries we should have,” O’Neil said. “We determine what the dials are in the cockpit, starting with the algorithmic audit. We ask, ‘what could go wrong?’”
State and local governments are starting to demand fairer AI systems. Colorado’s new law, which goes into effect on Nov. 14, requires life insurance companies that use AI tools to implement policies that prevent discrimination. New York City requires companies that are using AI systems in hiring to conduct independent audits to assess biases.
Most of O’Neil’s work involves figuring out what problems to measure and how to measure them effectively. She investigates whether algorithms produce bad outcomes for protected groups and then presents her findings to regulators and lawyers, who decide whether the discrimination is legal.
Anticipating AI Risks and Impacts
The European Union (EU) is spearheading AI legislation with its proposed AI Act. While the framework likely won’t be enforced until 2026, it states that high-risk AI systems (which pose significant risks to human health, safety, or fundamental rights) must identify and analyze “known and foreseeable risks.”
“This is not about sci-fi,” he said. “It's not about predicting exactly what's going to happen. It's about rigorously laying out some plausible or possible realities or paths that the technology could take.”
Surveying a diverse set of people with knowledge about a field or topic can help. Diakopoulos’ ongoing research with CASMI is exploring how to develop a method that anticipates the impacts of new AI technologies. As part of this work, Kimon Kieslich, University of Amsterdam faculty of law, and Natali Helberger, University of Amsterdam professor in law and digital technology, co-wrote a research paper with Diakopoulos that asked news consumers, technology developers, and content creators in EU member states to write about how generative AI can impact the news environment.
“This is still a work in progress, but as we move this kind of method into the US context, we’ll be looking for ways to enhance demographic diversity and better understand how that intersects with people's conception of impact,” Diakopoulos said.
“Ultimately, the underlying point is that we’re going to need lots of different perspectives from lots of different cognitively diverse individuals with different backgrounds, experiences, and sets of expertise,” he added. “It’s going to enable better foresight.”
The workshop’s fireside chat addressed challenges in anticipating issues and the need for structure in the commercial world. Its participants were Stevie Bergman, Google DeepMind senior research scientist; Zachary Lipton, chief scientific officer for medical startup Abridge; and Nicol Turner Lee, The Brookings Institution senior fellow in governance studies and director of the Center for Technology Innovation.
Laying the Groundwork to Develop a Testbed
A main focus of the workshop was to lay the groundwork to develop a testbed for evaluating the risks and impacts of AI. To do this, participants were divided into groups to discuss four topics: 1) turning non-numerical information gathered through interviews and observations (qualitative methods) into measurable data (quantitative metrics); 2) testing applications, models, or systems using sociotechnical methods; 3) designing a sociotechnical testbed; and 4) establishing robust and reusable documentation standards.
The first group discussed developing concrete processes and guidelines for diverse participation, evaluating processes using workshops or the NIST testbed, incentivizing people to participate through regulation or professional standards, and raising public awareness via blogs and investigative journalism.
The second group considered the importance of research funding, shifting the culture toward a human-centered focus, integrating sociotechnical perspectives, using shared language, and experimenting boldly towards solutions.
The third group talked about communication, developing a sustainable and profitable testbed, creating a community of practice, and building a pilot study ‒ a key objective. Piloting would involve creating metrics, recruiting people, questioning assumptions, scoping contexts, and, above all, just getting something done.
The fourth group discussed aligning on the purpose of documentation, crafting an implementation plan (which includes measurement), establishing minimum standards for what must be developed, piloting documentation methods (such as a regulatory sandbox) before scaling them, and having public engagement for education and feedback.
CASMI will use these findings to deliver a report to NIST.
“It was a productive workshop,” Hammond said. “We’ve done something here that’ll be a great foundation and will move this process forward.”
CASMI convenes workshops to enhance its mission of operationalizing a safety science in AI.