Moving Closer to Efficiently Using Earth’s Power
Chris Wolverton proposed way to unify heat carriers to estimate lower limit of lattice thermal conductivity
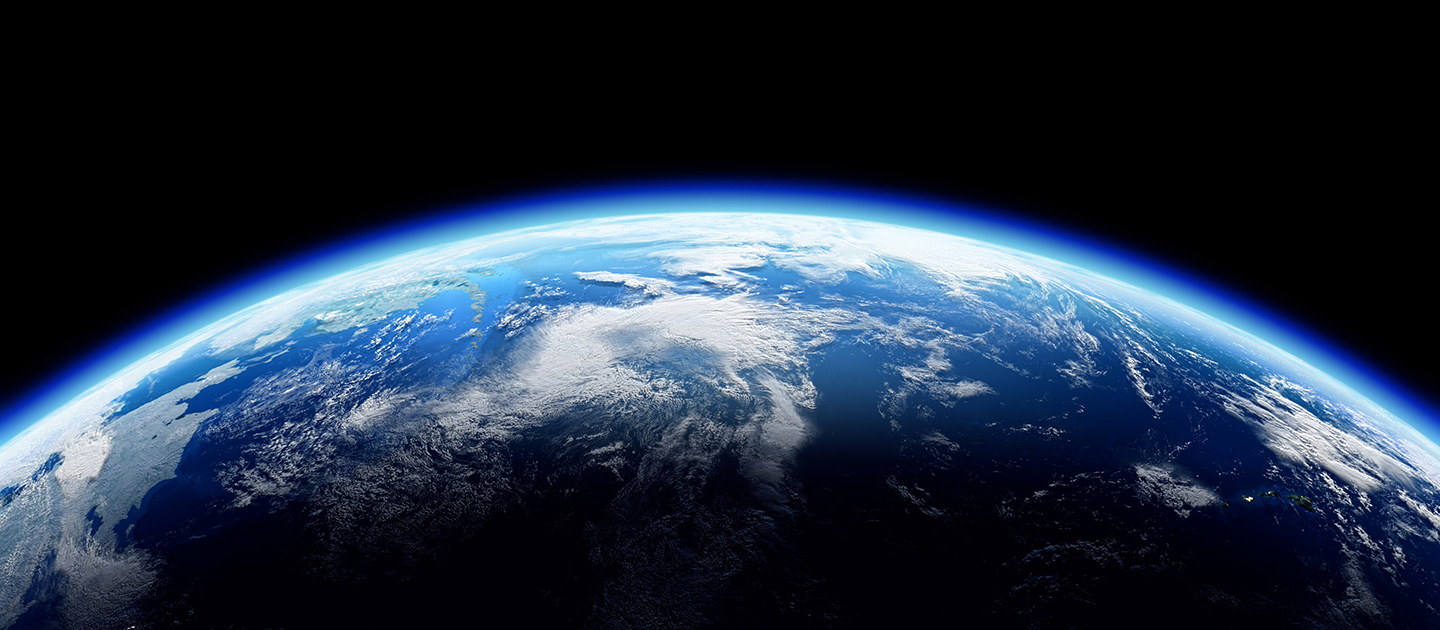
The planet Earth produces the same amount of heat as several thousand nuclear power plants. This thermal energy could be put into use in many ways, but there’s a significant problem:
Two-thirds of that energy is lost.
Finding a way to close that gap has been a pressing challenge for scientists for the better part of the last 100 years. Models have been produced by some of the world’s leading minds, including Albert Einstein, and those blueprints have been built upon but never solved the issue.
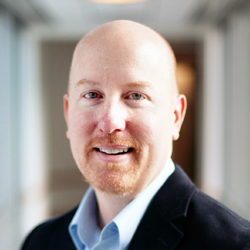
A recent paper by Northwestern Engineering’s Chris Wolverton could help the field come closer to finding answers.
Lattice thermal conductivity, a fundamental property of materials, plays a vital role in various technological applications, including thermal energy conversion and management. Understanding its lower limit – known as its minimum thermal conductivity – is crucial because that knowledge helps understand the material’s capabilities, but challenging. Wolverton and his teammates proposed a model that unifies different kinds of heat carriers to model the lower limit of lattice thermal conductivity and applies it to thousands of inorganic compounds.
And to ensure accuracy on a large scale, the investigators built a machine-learning model that has the potential to open the pathway to rational design of materials with a low thermal conductivity.
This system allows scientists to search very efficiently for new materials that have low thermal conductivity, which could potentially change the balance of how much energy is used and how much is wasted. Its use of the growing field of artificial intelligence moves researchers closer to using its capabilities to predict which materials have the best thermal conductivity.
“If you could somehow convert a fraction of that heat into useful electrical work, it would be a game-changer,” Wolverton said.
“If you give a computer thousands and thousands of examples of a chemical formula and the thermal conductivity, the question is, can it learn the relationship between those two? It's a very, very complicated relationship, but if you give it many examples, can it learn that? If it can, then it can predict the relationship for new compositions where you don’t already know the thermal conductivity.”
“The work introduces machine learning models that have the unique capability to analyze solids with both structural and compositional disorder,” said lead author Yi Xia, currently an assistant professor of mechanical and materials engineering at Portland State University and a postdoc at Northwestern when the research was conducted. “This universally applicable model allows researchers to quickly estimate the lattice thermal conductivity in amorphous solids, which holds significant implications for the design of thermal insulating materials. This advancement facilitates the development of innovative solutions in the field of thermal insulation.”
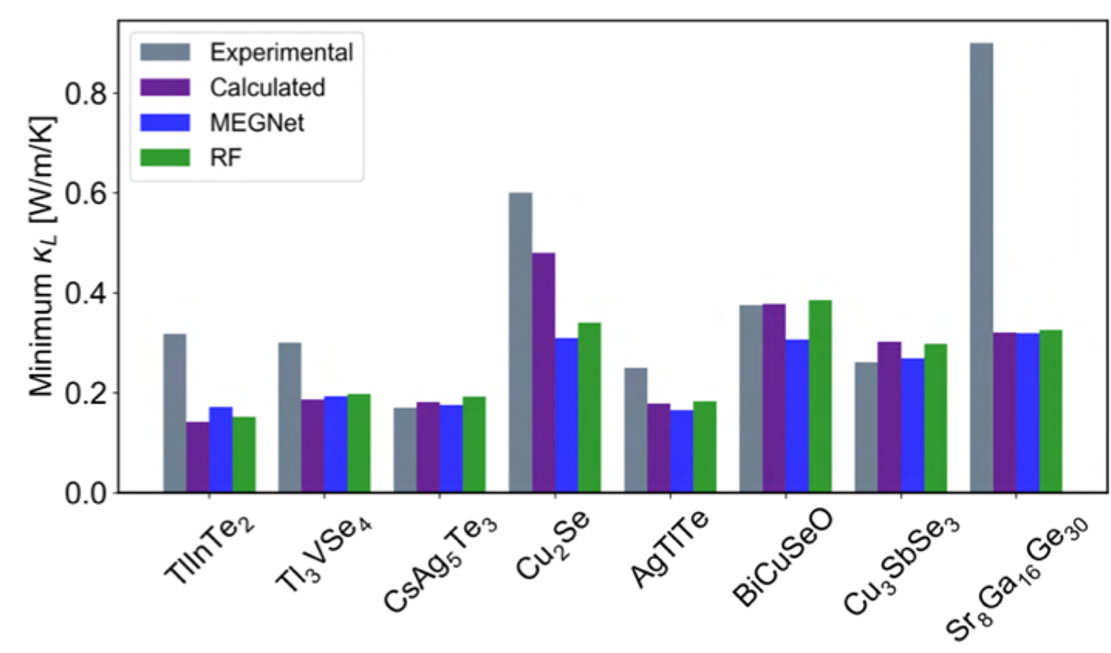
The work was presented in “A Unified Understanding of Minimum Lattice Thermal Conductivity,” published June 20 in the Proceedings of the National Academy of Sciences. Wolverton is a professor of materials science and engineering at the McCormick School of Engineering. The team also included Mercouri Kanatzidis, Charles E. and Emma H. Morrison Professor of Chemistry and (by courtesy) Professor of Materials Science and Engineering at Northwestern; Dale Gaines II, a materials science PhD candidate in Wolverton’s lab; Zhi Li, a postdoctoral fellow in materials science and engineering in Wolverton’s lab; Jiangang He from the University of Science and Technology Beijing; Koushik Pal from Los Alamos National Laboratory; and Vidvuds Ozoliņš from Yale University.
As Xia said, the model allows researchers to calculate the minimum thermal conductivity for a whole variety of compounds, upwards of 2,000. Using the machine-learning model, Wolverton and his colleagues predicted the minimum thermal conductivity of materials and compared them to the experimentally measured values. In some cases, they found similar totals.
Wolverton said that means, experimentally for those materials, they are already pretty much at the minimum.
“There's basically nothing left to do anymore to try to lower the thermal connectivity, and you might as well focus on other properties for those materials,” Wolverton said. “But with some materials, we find there's a big gap between the measured connectivity and this minimum, and that implies that there is room to tailor these materials, and to try and find ways to lower their thermal connectivity. It separates materials into ones where there's really promise, and maybe ones where we've kind of already exploited everything we can.”