New Method Could Allow Better Evaluation of Cells
Novel process offers improvement to analysis, bringing increasingly confident biological results
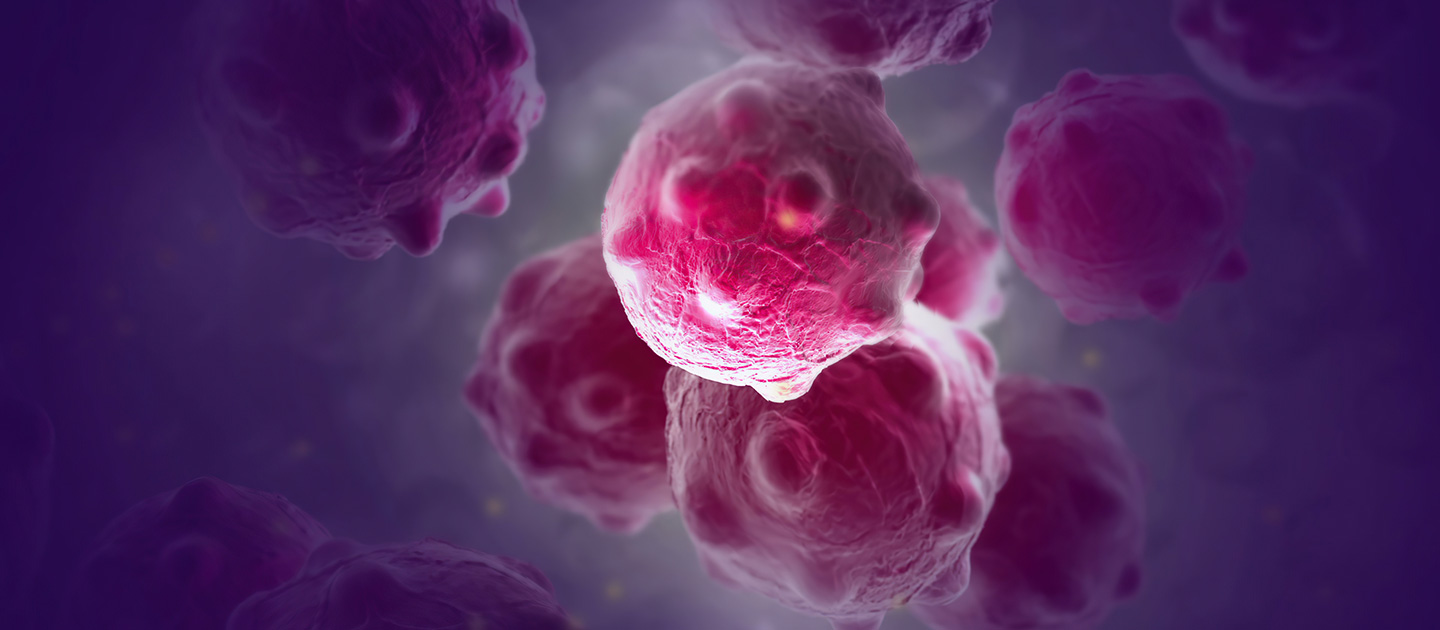
“Single-cell omics” is producing exciting biological data. The field measures how active every gene is in every cell of an organism and promises to yield valuable information on how our bodies work. One example is how a single-cell measurement of a cancer tumor might reveal which genes are responsible for the disease, potentially allowing the disruption or prevention of the disease.
However, these data sets are incredibly difficult to analyze because of the amount of information and their complexity, so any improvements to the analysis methods will necessarily improve the quality and magnitude of the results of these experiments.
A team led by Northwestern Engineering’s Madhav Mani developed a new method that offers a general and direct improvement to a broad class of analysis techniques that could lead to increasingly confident biological results.
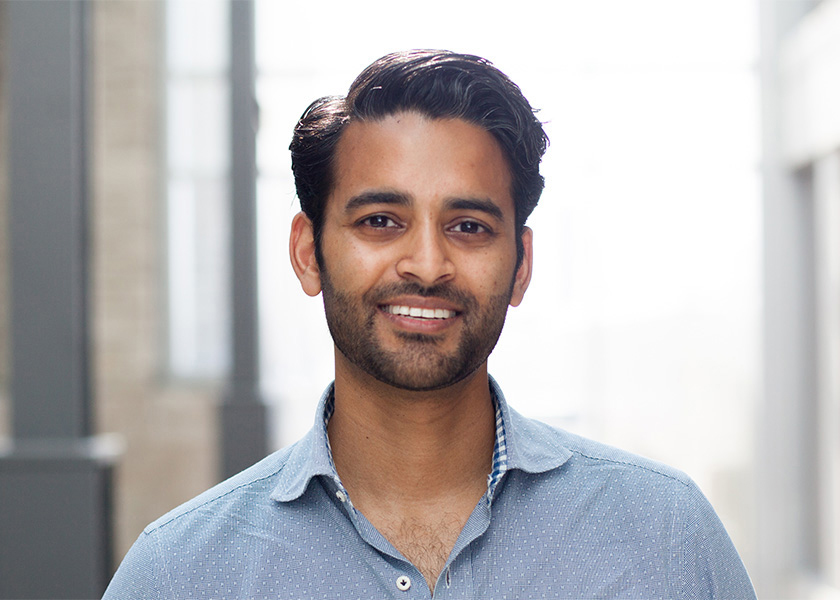
Mani and his colleagues presented the new method in the paper “EMBEDR: Distinguishing Signal from Noise in Single-Cell Omics Data,” published February 8 in the academic journal Patterns, a subjournal of Cell. Mani, assistant professor of engineering sciences and applied mathematics at the McCormick School of Engineering, was the paper’s corresponding author. ESAM professor William Kath, Margaret Fuller Boos Professor and professor of engineering sciences and applied mathematics, was a co-author. Eric Johnson, a PhD student in Kath’s lab, was the study’s first author.
Known as Empirical Marginal Resampling Better Evaluates Dimensionality Reduction, (EMBEDR), the process addresses the crisis of confidence researchers have in interpreting powerful datasets, which sometimes can resemble Rorschach inkblots and require special care to ensure that the supposed patterns are not just noise.
“Data analysis is increasingly important to biological research, but while biologists are constantly inventing amazing new technologies, the mathematics and statistics in some areas have not reached a similar level of maturity,” Mani said. “We sought to take a wider view of the specific challenges facing biological data analysis, and showed how we developed EMBEDR based on pre-set guiding principles.”
By applying a statistical and data-driven approach, Mani and his team found that EMBEDR results in more confident, trustworthy, and consistent results than current methods when applied to real data sets. More specifically, EMBEDR is a tool for assessing the quality of dimensionality reduction algorithms, which are data analysis methods used to condense large data sets into smaller, more interpretable representations. EMBEDR calculates a quality score for each sample in the data set, which allows a researcher to understand what part of the reduced dataset is consistent with patterns in the raw data.
The researchers then use these quality scores to perform common tasks such as cell-type clustering and gene enrichment analysis. They also show that using EMBEDR generates a completely novel spectral view of the data, which is used to develop an improved dimensionality reduction method that is considerably more stable and interpretable than current methods.
This information and these new tools could improve the quality and confidence of new biological results generated from single-cell experiments.
We hope that presenting our work this way will facilitate more theoretical discussions in future work as well as invite non-specialists to think about the challenges of data analysis. Assistant Professor of Engineering Sciences and Applied Mathematics
“We hope that presenting our work this way will facilitate more theoretical discussions in future work as well as invite non-specialists to think about the challenges of data analysis,” Mani said. “The framework for assessing quality suggested by EMBEDR is presented very generally; we focused on applying the method to single-cell datasets where these analysis methods are most often used, but we hope that EMBEDR also inspires new data analysis methods outside of biology.”
The method was inspired by several topics taught in the ESAM graduate-level course “What Do Your Data Say?” that introduces students to modern data analysis tools. The class explores how computers can be used to simulate data or iteratively refine information when researchers are faced with problems that don’t have written or analytical answers, a technique that was directly incorporated into EMBEDR.
While EMBEDR is a starting point, the researchers wanted to deliver a proof of concept of their framework. With this established, they hope to apply EMBEDR in other collaborations to generate new biology.
“This work is really exciting because it is very different from a lot of the other work in the field,” Mani said. “We really tried to comprehensively examine the existing methodologies before starting ground-up with a new method that takes the best practices from all areas. The paper uses ideas from several disciplines in statistics, computer science, and mathematics. We welcome suggestions for improvements or extensions.”
The platform can be accessed here.