New Transistors Spike Like Neurons
Made from nanomaterials, device can perform several different classes of spiking
Neuromorphic computing — where computers function like human brains to perform complex tasks much more efficiently than current computers — holds promise for breakthroughs in artificial intelligence and machine learning.
But such computers can be difficult to create with traditional silicon technology. Northwestern Engineering researchers have taken a step toward realizing neuromorphic computing by creating a new transistor from nanomaterials that can generate voltage “spikes” like a neuron.
“In order to mimic the brain, we need to mimic the function of neurons,” said Mark Hersam, Walter P. Murphy Professor of Materials Science and Engineering and lead author of the research. “This goal can be achieved using traditional silicon technology, but we show a much simpler means of achieving neuron function, which is needed to increase the density of artificial neurons on a chip.”
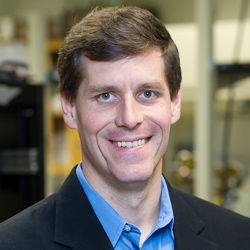
Mixing nanomaterials in neuron-like devices
Neurons transmit information in the brain through an action potential, which is a spike in voltage that leads to communication with other neurons. While researchers have mimicked action potentials with traditional silicon transistors, it has required complicated circuits that require a dozen or more devices.
As they approached their new research, Hersam and his group hypothesized that they could create a simpler spiking circuit if they had the ability to tune the height, peak position, and width of a device’s Gaussian response — the bell curve that shows the distribution of its output current.
To do this, they created a device based on two different nanomaterials — molybdenum disulfide and carbon nanotubes — along with two gate electrodes. These nanomaterials are strongly affected by applied electric fields, while the two gates allowed improved electric field control compared to previous designs. By placing one gate on top, followed by offset layers of the two nanomaterials and a gate on the bottom, they developed a device that had several degrees of tunability.
“This device has the potential for more sophisticated functionality that a standard silicon transistor,” Hersam said. Not only can it spike like a neuron, it can perform several different classes of spiking that different neurons perform in different areas of the brain, like continuous spiking or phasic spiking.
“Normally you would have to redesign a circuit to get each spiking behavior,” Hersam said. “However, this device is so flexible and tunable that we can achieve a dozen spiking responses based on how we control the voltage.”
Combining technology with memtransistors
Previously, Hersam and his group took a step forward in neuromorphic computing by developing a “memtransistor” — a combination transistor and memristor that efficiently achieves brain-like function by performing both memory and information processing. The memtransistor also possesses multiple terminals that allow the high degrees of interconnectivity that are present in biological neural networks.
The new spiking device does not possess multiple terminals, so Hersam and his team hope to next integrate the two devices in the future to combine their complementary attributes.
“We also want to make these devices even smaller and more compact,” he said. “In this manner, we can increase the device density and achieve even more sophisticated neuromorphic function.”
Future of neuromorphic computing requires collaboration
Devices like these, which use a combination of nanomaterials, are likely going to be the way that we develop computers that better mimic the brain, Hersam argued in the Nature Nanotechnology review paper.
Neuromorphic computing is needed if we want to advance processes like artificial intelligence and machine learning, he says. While traditional computers are successful at some tasks, they don’t compare to the brain in many others. Not only does the brain seamlessly combine memory and information processing— two processes that are separate in today’s computers — it does so with much less energy than conventional digital computing.
“The amount of energy consumption by conventional server farms is becoming a substantial fraction of the overall grid,” Hersam said. “Neuromorphic computing has the potential to significantly decrease energy demand.”
Although the review paper comprehensively highlights the many ways that scientists are trying to achieve neuromorphic computing, Hersam believes that a bottom-up approach — using the neuron as inspiration for devices — is the right way forward.
Not only that, “Close collaboration between material scientists, neuroscientists, and computer architects is going to be crucial,” he wrote.
Northwestern Engineering professors are currently approaching neuromorphic computing from several different angles. James Rondinelli, Morris E. Fine Junior Professor in Materials and Manufacturing, is designing novel materials platforms for use in memristor devices. Jie Gu, assistant professor of electrical and computer engineering, is using neuromorphic computing to accelerate emerging machine learning tasks on conventional integrated circuit chips for low-power mobile or Internet of Things devices. Gokhan Memik, professor of electrical and computer engineering, has been working on architectural aspects of ReRAM-based neural network accelerators.
“Northwestern Engineering is truly taking a comprehensive, whole-brain approach to the problem of neuromorphic computing,” Hersam said.
The Nature Communications research was funded by the National Science Foundation, National Institute of Standards and Technology, and Office of Naval Research. Other authors on the paper include postdoctoral researchers Vinod K. Sangwan and Hocheon Yoo; graduate students Megan E. Beck, Silu Guo William A. Gaviria Rojas, Hadallia Bergeron, and Ahish Shylendra; undergraduate student Katherine Su; and Professor Amit R. Trivedi of the University of Illinois at Chicago.