New Research Uses Bayesian Cognition Approach to Improve Data Visualization
Professor Jessica Hullman and collaborators apply a formal model of belief updating to improve the design and evaluation of data visualizations
News consumers often encounter visualizations through data journalism about topics like climate change or election results – topics where people bring their own background knowledge and beliefs when assessing the information.
These biases can affect how people interpret the data, and consequently how they update their existing beliefs based on the new information. Yet designers of visualizations and other data presentations typically do not consider these factors when creating visualizations. New research from Jessica Hullman, Breed Junior Professor of Design and assistant professor of computer science and journalism, utilizes formal models to analyze how people interpret and update their beliefs based off data visualizations.
Northwestern Engineering’s Hullman and her students and collaborators at the University of Washington use Bayesian models of cognition by eliciting people’s beliefs before and after showing them data. They then compare how much they actually change their beliefs based on the data to the predictions of the Bayesian model, which says how much they should change their beliefs if they are rational processors of information.
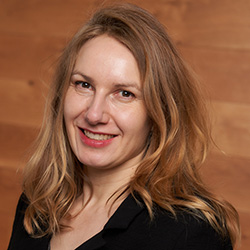
Her paper on this topic, “A Bayesian Cognition Approach to Improve Data Visualization,” along with three other papers from her lab, was accepted for the Association for Computing Machinery (ACM)’s CHI Conference on Human Factors in Computing Systems, the premier international conference on Human-Computer Interaction (HCI). CHI 2019 will be held in Glasgow, Scotland, from May 4-9, 2019, and the paper will be archived in ACM SIGCHI’s Conference Proceedings.
Beyond helping data journalists better convey data visualizations, these models and the associated methods for eliciting beliefs could also apply to human-in-the-loop artificial intelligence or data analysis systems, which aim to combine the knowledge of humans and systems to enable forms of reasoning that neither could do alone.
This research was funded by Hullman’s CAREER Award, “Enhancing Critical Reflection on Data by Integrating Users' Expectations in Visualization Interaction.”
The three other papers coauthored by Hullman and accepted for the CHI 2019 conference are:
- Decision-Making Under Uncertainty in Research Synthesis: Designing for the Garden of Forking Paths: an interview study of people who do systematic review of prior research experiments to evaluate the overall true effect of the research
- Vocal Shortcuts for Creative Experts: developing speech-based interfaces to help creative professionals as they use authoring tools like Adobe Photoshop
- Some Prior(s) Experience Necessary: helping HCI researchers who are not statistical experts learn Bayesian statistics