Faculty DirectoryHan Liu
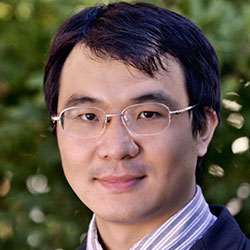
Orrington Lunt Professor of Computer Science and (by courtesy) Industrial Engineering & Management Sciences
Professor of Statistics
Contact
2233 Tech DriveMudd Room 3119
Evanston, IL 60208-3109
Email Han Liu
Website
Departments
Education
Ph.D. Machine Learning and Statistics, Carnegie Mellon University, Pittsburgh, PA
Biography
Han Liu directs the MAGICS (Modern Artificial General Intelligible and Computer Systems) lab at the Northwestern University. He has been the director of the deep reinforcement learning center at Tencent AI Lab and had been a professor at the Princeton University and Johns Hopkins University. He received a joint PhD in Machine Learning and Statistics from the Machine Learning Department at the Carnegie Mellon University, advised by John Lafferty and Larry Wasserman. His research lies at the intersection of artificial intelligence and computer systems, which deploys statistical machine learning methods on edges and clouds to achieve analytical advantages. Han Liu has received numerous research awards including the Alfred P Sloan Fellowship in Mathematics, the IMS Tweedie New Researcher Award, the ASA Noether Young Scholar Award, the NSF CAREER Award, the Howard B Wentz Award and the Umesh Gavaskar Memorial Best Dissertation Award. He is now serving as associate editors for the Journal of American Statistical Association, the Electronic Journal of Statistics, the Technometrics, and the Journal of Portfolio Management.
Research Interests
Han Liu directs the MAGICS(Modern Artificial General Intelligible and Computer Systems) lab at Northwestern University. He is also the director of the Center for Foundation Models and Generative AI (CFMG) at Northwestern University. He has been the director of the deep reinforcement learning center at Tencent AI Lab and has been a professor at Princeton University and Johns Hopkins University. He received a joint PhD in Machine Learning and Statistics from the Machine Learning Department at Carnegie Mellon University, advised by John Lafferty and Larry Wasserman. His research integrates modern artificial intelligence and computer systems, which exploits large foundation models and probabilistic graphical models to revolutionize science, engineering, and business. Han Liu has received numerous research awards including the Presidential Early Career Awards for Scientists and Engineers, the Alfred P Sloan Fellowship in Mathematics, the IMS Tweedie New Researcher Award, the ASA Noether Young Scholar Award, the NSF CAREER Award, the Howard B Wentz Award and the Umesh Gavaskar Memorial Best Dissertation Award. He has been associate editors for the Journal of American Statistical Association, the Electronic Journal of Statistics, the Technometrics, and the Journal of Portfolio Management. He has also been seriving as area chairs for NeurIPS, ICML, ICLR conferences.
Selected Publications
- Hu, Jerry Yao Chieh; Lin, Thomas; Song, Zhao; Liu, Han, On Computational Limits of Modern Hopfield Models, Proceedings of Machine Learning Research (2024).
- Hu, Jerry Yao Chieh; Chang, Pei Hsuan; Luo, Haozheng; Chen, Hong Yu; Li, Weijian; Wang, Wei Po; Liu, Han, Outlier-Efficient Hopfield Layers for Large Transformer-Based Models, Proceedings of Machine Learning Research (2024).
- Xu, Chenwei; Huang, Yu Chao; Hu, Jerry Yao Chieh; Li, Weijian; Gilani, Ammar; Goan, Hsi Sheng; Liu, Han, BiSHop, Proceedings of Machine Learning Research (2024).
- Wu, Dennis; Hu, Jerry Yao Chieh; Hsiao, Teng Yun; Liu, Han, Uniform Memory Retrieval with Larger Capacity for Modern Hopfield Models, Proceedings of Machine Learning Research (2024).
- Cao, Yuan; Neykov, Matey; Liu, Han, High-temperature structure detection in ferromagnets, Information and Inference (2022).
- Lu, Junwei; Han, Fang; Liu, Han, Robust Scatter Matrix Estimation for High Dimensional Distributions with Heavy Tail, IEEE Transactions on Information Theory 67(8):5283-5304 (2021).
- Ma, Cong; Lu, Junwei; Liu, Han, Inter-Subject Analysis, Journal of the American Statistical Association 116(534):746-755 (2021).
- Tan, Kean Ming; Lu, Junwei; Zhang, Tong; Liu, Han, Estimating and inferring the maximum degree of stimulus-locked time-varying brain connectivity networks, Biometrics 77(2):379-390 (2020).
- Lin, Kevin Z.; Liu, Han; Roeder, Kathryn, Covariance-Based Sample Selection for Heterogeneous Data, Journal of the American Statistical Association 116(533):54-67 (2020).
- Lu, Junwei; Kolar, Mladen; Liu, Han, Kernel Meets Sieve, Journal of the American Statistical Association 115(532):2084-2099 (2020).
- Rosenblum, Michael; Fang, Ethan X.; Liu, Han, Optimal, two-stage, adaptive enrichment designs for randomized trials, using sparse linear programming, Journal of the Royal Statistical Society. Series B: Statistical Methodology 82(3):749-772 (2020).
- Ge, Jason; Li, Xingguo; Jiang, Haoming; Liu, Han; Zhang, Tong; Wang, Mengdi; Zhao, Tuo, Picasso, Journal of Machine Learning Research 20 (2019).
- Tan, Kean Ming; Lu, Junwei; Zhang, Tong; Liu, Han, Layer-wise learning strategy for nonparametric tensor product smoothing spline regression and graphical models, Journal of Machine Learning Research 20 (2019).
- Neykov, Matey; Liu, Han, Property testing in high-dimensional ising models, Annals of Statistics 47(5):2472-2503 (2019).
- Wang, Qing; Xiong, Jiechao; Han, Lei; Sun, Peng; Liu, Han; Zhang, Tong, Exponentially weighted imitation learning for batched historical data, Advances in Neural Information Processing Systems 31:6288-6297 (2018).
- Eisenach, Carson; Liu, Han, Efficient, certifiably optimal clustering with applications to latent variable graphical models, Mathematical Programming 176(1-2):137-173 (2019).
- Yang, Zhuoran; Ning, Yang; Liu, Han, On semiparametric exponential family graphical models, Journal of Machine Learning Research 19:1-59 (2018).
- Neykov, Matey; Lu, Junwei; Liu, Han, Combinatorial inference for graphical models, Annals of Statistics 47(2):795-827 (2019).
- Zhou, Wen Xin; Bose, Koushiki; Fan, Jianqing; Liu, Han, A new perspective on robust M-estimation, Annals of Statistics 46(5):1904-1931 (2018).
- Li, Xingguo; Lu, Junwei; Arora, Raman; Haupt, Jarvis; Liu, Han; Wang, Zhaoran; Zhao, Tuo, Symmetry, Saddle Points, and Global Optimization Landscape of Nonconvex Matrix Factorization, IEEE Transactions on Information Theory 65(6):3489-3514 (2019).
- Tan, Kean Ming; Wang, Zhaoran; Zhang, Tong; Liu, Han; Cook, R. Dennis, A convex formulation for high-dimensional sparse sliced inverse regression, Biometrika 105(4):769-782 (2018).