Why Machine Learning Could Change Experimental Solid Mechanics
A team with Horacio Espinosa highlighted how ML is showing promise in the field
In the field of experimental solid mechanics – a discipline that focuses on understanding and measuring the physical properties of different materials – researchers have traditionally relied on physical experiments and computational models to study and design materials.
A new review paper from a team led by Northwestern Engineering’s Horacio Espinosa, however, highlights how applying machine learning (ML) to the field is producing promising results.
The new approach could be crucial in the development of materials used to build the cars and smartphone screens of the future.
“The future of materials science is being shaped by machine learning and deep learning, and this could lead to impressive advancements in the materials we use every day, from the steel in our buildings to the glass on our smartphones,” said Espinosa, the James N. and Nancy J. Farley Professor in Manufacturing and Entrepreneurship at the McCormick School of Engineering.
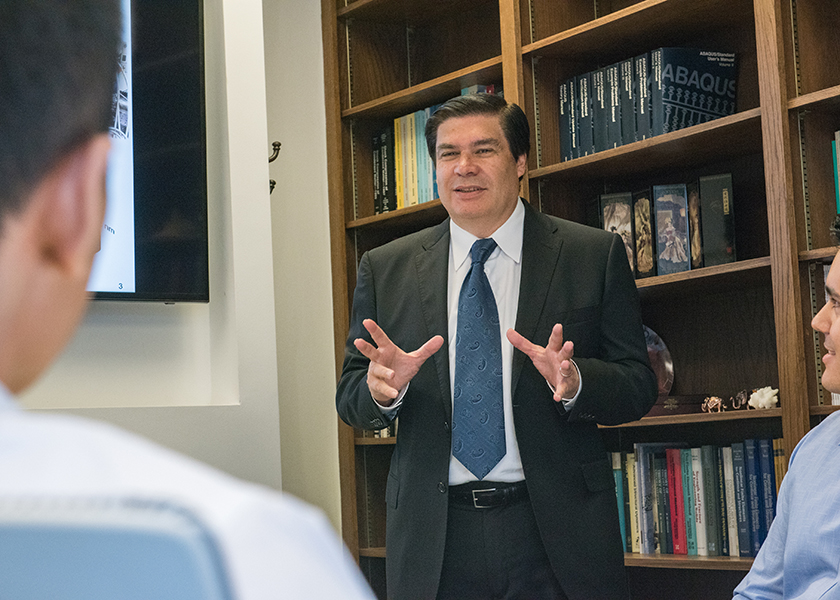
Espinosa and his colleagues highlighted their work in “Recent Advances and Applications of Machine Learning in Experimental Solid Mechanics: A Review,” published July 28 in the academic journal Applied Mechanics Reviews. The paper’s coauthors include Hanxun Jin, currently a postdoc at the California Institute of Technology and a former postdoctoral researcher at Northwestern under Espinosa; and Enrui Zhang, who earned a PhD in applied mathematics from Brown University.
In their paper, the team emphasized the multidisciplinary approach required to harness the potential of ML in experimental solid mechanics. While their review focused on the experimental aspects, the researchers stress that it’s the integration of computational and theoretical mechanics, materials science, and other fields such as data science that will provide the most meaningful advancements.
“The use of machine learning in this field doesn't replace traditional methods but augments them,” Zhang said. “The technology is not without its challenges or faults, such as reliability or noisy data, but the integration of machine learning can help in extrapolating findings from computational models and physical experiments to make predictions about new, untested scenarios.”
The team argues that integrating ML in solid mechanics could have a positive impact in many ways.
One example is speeding up the material design process by creating innovative materials with superior properties faster than before. ML could also help researchers manage uncertainties in experimental data, leading to better predictions about material properties and, subsequently, safer, and more reliable products in industry. New experimental techniques, the team writes, could be inspired by ML because of how much data it can generate and analyze.
“This might lead to discoveries of material properties we weren't aware of before,” Jin said.
The authors also propose several future research directions. They call for the integration of ML in experimental solid mechanics, showing how it has been used in a wide range of applications, from constructing surrogate models for constitutive modeling to extracting unknown mechanical parameters and designing architected materials. The team also suggests the employment of methods like Bayesian analysis and deep ensembles to quantify and manage uncertainties from both experimental data and ML architectures. Finally, the authors speculate that ML could significantly enhance the experimental design data process, potentially identifying material properties that might otherwise remain concealed and inspiring the development of novel experimental techniques capable of generating big data with high information content.