Atomistic Experiments and Machine-Learning-Inspired Modeling Lead to Better Understanding of 2D Materials
A team of investigators highlighted advances in atomic-scale experimentation
The global market for 2D materials — already estimated at several billion dollars annually — is growing at a 4 percent rate. This is explained by the importance of these newly synthesized materials, with applications ranging from energy to optics, electronics, catalysis, bio-detection, and structural materials.
Advancing models and experiments can enable the design of 2D materials that more reliably support devices and systems, which could impact the semiconductor, automotive, pharmaceutical, and energy industries. New work from Northwestern Engineering researchers takes another step toward better evaluating 2D materials.
A team of investigators, led by Professor Horacio Espinosa, reported advances in experimentation and machine-learning-inspired modeling for the evaluation of 2D materials. Espinosa and his colleagues performed in situ high-resolution transmission electron microscopy atomistic measurements, directly comparing the atomic structures and material toughness of 2D materials with those same qualities predicted by molecular dynamics simulations using inter-atomic potentials based on ab initio data.
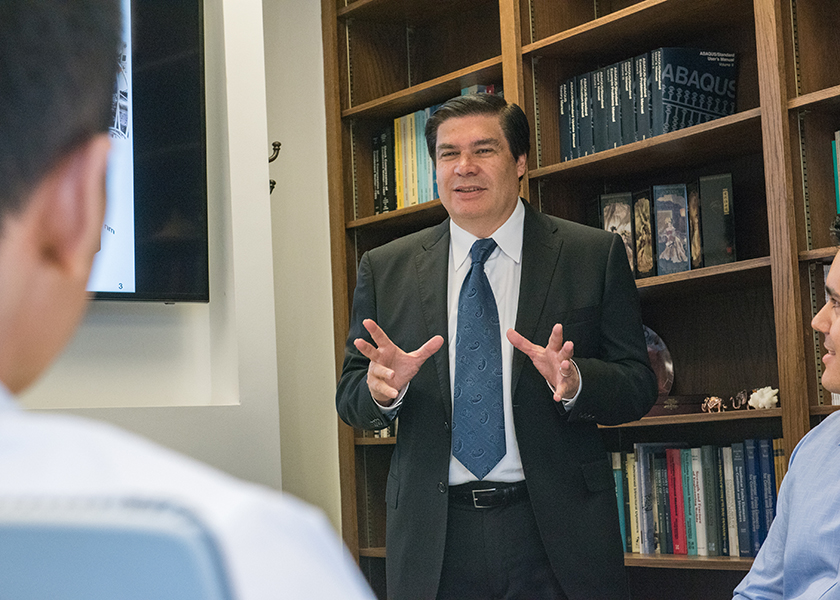
“The integrated framework provides a robust approach to obtaining intrinsic mechanical properties of 2D materials — in their pristine and defective states — and informs the analysis of device reliability with unprecedented accuracy,” Espinosa said.
Using this method, the simulations can accurately capture energy levels and atomic deformations near a crack tip — where bond breaking and other failure mechanisms depend on the material’s chemical composition.
This process represents a step forward and promises to help researchers achieve more precise results.
“The higher accuracy achieved with our integrated framework, in comparison to previous literature reports, enables around 150 times fewer errors in the fatigue life prediction of nanodevices fabricated from pristine 2D materials,” Espinosa said.
Espinosa is the James N. and Nancy J. Farley Professor of Manufacturing and Entrepreneurship at the McCormick School of Engineering. The work was explained in the paper “Atomistic Measurement and Modeling of Intrinsic Fracture Toughness of Two-Dimensional Materials,” published Nov. 4 in the Proceedings of the National Academy of Sciences.
The promise of computational material design is based on atomistic models with predictive capabilities. While quantum chemistry simulations can be used during unit cell property screening of 2D materials, device-level analysis requires large-scale atomistic simulations employing interatomic potentials. In this context, there is a need for continuous advances in inter-atomic force description, for example, via active machine learning and atomistic experiments that can provide measurements of quantities of interest such as mechanical and thermal properties.
This work, building off 2021 research that showed a multi-objective parametrization of interatomic potentials for large deformation pathways and fracture of two-dimensional materials, will be further explored to develop predictive atomistic models capable of describing thermomechanical properties.
In another effort in collaboration with researchers at Drexel University, the team will continue this work by investigating carbides and nitrides of transition metals, known as MXenes, a more complex and fast-growing family of 2D materials with applications to electromagnetic shielding, energy storage, biosensing, and structural composites.
This work was funded by the National Science Foundation (grant CMMI 1953806), the Office of Naval Research (Grant N00014-22-1-2133), and the US Department of Energy’s Office of Science and Office of Basic Energy Sciences (Contract No. DE-AC02-06CH11357).