Textbook Introduces Students to Mechanistic Data Science
The book introduces a methodology for combining data science tools with mathematical scientific principles
A new textbook from three Northwestern Engineering faculty members highlights an exciting new way to solve problems using the growing discipline of data science.
Coauthored by Wing K. Liu, Zhengtao Gan, and Mark Fleming, Mechanistic Data Science for STEM Education and Applications (Springer, 2021) introduces mechanistic data science (MDS) as a structured methodology for combining data science tools with mathematical scientific principles to solve intractable problems.
Traditional data science methodologies require copious quantities of data to show a reliable pattern, but the amount of required data can be greatly reduced by considering the mathematical science principles. These data science and mechanistic analysis steps are presented in an intuitive manner that emphasizes practical concepts for solving problems such as tracking the spread of COVID-19 and other contagious viruses, developing autonomous vehicles, and improving computer vision.
“The whole idea is that I can teach a student on all levels,” Liu said. “We use MDS to integrate the concepts of STEM together.”
Liu is Walter P. Murphy Professor of Mechanical Engineering and Civil and Environmental Engineering at the McCormick School of Engineering. Gan is a research assistant professor in the mechanical engineering department, while Fleming is a mechanical engineering adjunct professor.
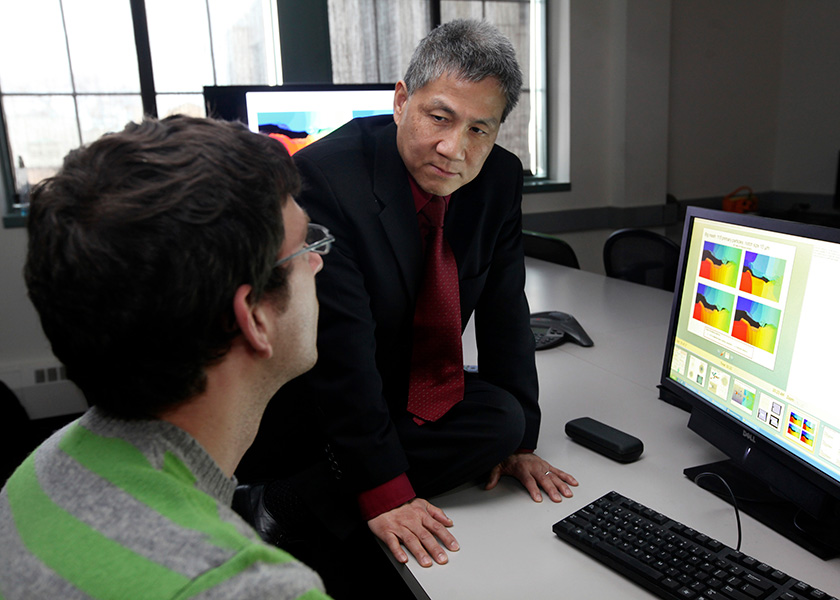
Presented in six modules, the entry-level textbook was written for engineering and data science undergraduate and graduate students, practicing scientists and engineers, as well as STEM high school students and teachers. The textbook focuses on three types of problems that MDS can help solve:
- Purely data-driven problems, a problem with abundant data but undeveloped or unavailable fundamental principles, typified by marketing behavior of people based on characteristics such as age and gender.
- Limited data and scientific knowledge problems, where neither the data nor the scientific principles provide a complete solution. This type of problem is typified by biomechanical problems such as scoliosis progression, in which fundamental scientific principles can be used to compute the direction of bone growth, but data is required to characterize the effects of age and gender.
- Known mathematical science principles with uncertain parameters, which can be computationally burdensome to solve. Scientific knowledge is the fundamental understanding of the world which allows people to make predictions, which enables future technologies and new discoveries. This type of problem is typified by physics problems such as determining the actual spring stiffness and damping properties of a spring mass system based on data collected from multiple cameras at different angles.
Liu was inspired to write the book in 2019 when he hosted summer tutorials, lectures, and research experiences on MDS for a small group of Northwestern undergraduates and the STEM Professionals As Resource Knowledge (SPARK) program at Stevenson High School in Lincolnshire, Illinois.
A year later, Liu initiated the course MECH_ENG 329: Mechanistic Data Science for Engineering, which introduces mechanistic data science for engineering through the integration of scientific knowledge, such as physics and mechanics, through the six basic data science concepts that became the foundational modules of the textbook: multimodal data generation and collection, extraction of mechanistic features, knowledge-driven dimension reduction, reduced order surrogate models, deep learning for regression and classification, and system and design.
Liu combined fundamental calculus with other numerical methods to create the class.
“That’s the way I want to relay them together, so this might be a new way to teach,” Liu said. “I wanted to introduce students to ideas to get more excited about STEM. Not only that, I wanted to bring in examples from daily life.”
Meanwhile, Liu founded the international conference “Mechanistic Machine Learning and Digital Twin for Computational Science, Engineering and Technology, MMLDT-CSET.” Working closely with the University of California San Diego, the inaugural conference was held in 2021 and received a $99,500 National Science Foundation grant to fund high school students, undergraduates, and graduate students to attend the event.
Another conference is planned for 2023 at the University of Texas at El Paso.