New Textbook Explores Foundations, Modern Applications of Predictive Analytics
Textbook features exercises and projects that connect theory to practical applications
Statistical learning, the science of identifying patterns in data and using data to build models and make predictions, is receiving growing attention across industries. With the data science platform industry expected to reach $26 billion by 2027, businesses are relying on predictive analytics using statistical learning more than ever to make better, more informed decisions from the vast data available to them.
While this emphasis on data analytics has spurred new educational programs and research directions at the intersection of statistics and computer science, the roots of many of these new statistical learning methods date back more than a century.

Ajit Tamhane, professor of industrial engineering and management sciences at Northwestern Engineering, has written a new textbook, Predictive Analytics: Parametric Models for Regression and Classification Using R (Wiley, 2021), which systematically explores the classical foundations of statistical learning and illustrates its applications to current challenges in big data through case studies and projects.
Tamhane wrote the book more than eight years after he launched MSiA 401/IEMS 462-1: Predictive Analytics I, the first class in a two-part sequence. The first part presents the classical parametric methods, while the second focuses on the modern computer intensive nonparametric methods. As he developed his lecture notes, examples, and exercises, he saw an opportunity to bring them together as a textbook.
“I’m motivated by a love for organizing the knowledge in a particular area and presenting it to students in the clearest way possible and with good examples and illustrations,” said Tamhane, who has authored four books and edited two more. “This book fills the need for a comprehensive review of classical methods of statistical learning and their connections to modern methods with big data business applications.”
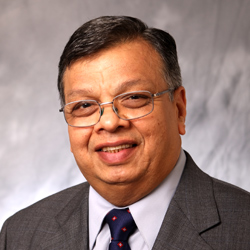
Predictive Analytics also features exercises based on moderate to large data sets. Using an open-source data analysis software, R, readers gain hands-on experience applying chapter themes to data sets from diverse fields including finance, marketing and sales, economics, education, engineering, and the sciences.
“The examples, exercises, and projects should be viewed as a vital complement to the text,” Tamhane said. “My hope is that when students take this approach to study the book, they will have understood the subject matter at a sufficient depth. They will be prepared to apply it in practice and go on to study at the next level, if they wish.”