Hartline Organizes The First Workshop on Algorithmic Game Theory and Data Science at FCRC'2015
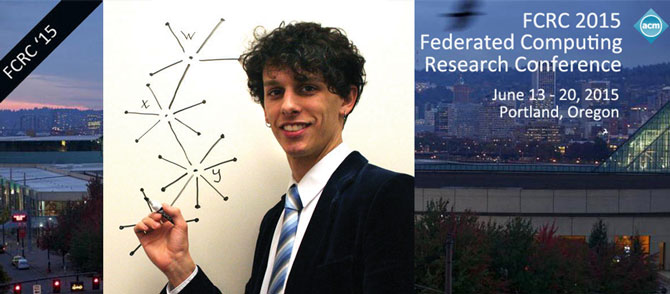
Prof. Jason Hartline organized The First Workshop on Algorithmic Game Theory and Data Science at the Federated Computing Research Conference (FCRC'2015) and in conjunction with the Sixteenth ACM Conference on Economics and Computation (EC'15) on June 15, 2015 in Portland, Oregon.
At this workshop, Prof. Hartline presented his work on "A/B Testing of Auctions" and EECS PhD Student Darrell Hoy exhibited his research on "Price of Anarchy from Data". The workshop was very well received, as well as highly attended and researchers and practitioners from academia and industry convene to discuss the burgeoning development in the intersection of Algorithmic Game Theory and Data Science.
Read Prof. Hartline's blog post previewing the workshop.
"A/B Testing of Auctions" Abstract: For many application areas A/B testing, which partitions users of a system into an A (control) and B (treatment) group to experiment between several application designs, enables Internet companies to optimize their services to the behavioral patterns of their users. Unfortunately, the A/B testing framework can not be applied in a straightforward manner to applications like auctions where the users (a.k.a., bidders) submit bids before the partitioning into the A and B groups is made. This paper combines auction theoretic modeling with the A/B testing framework to develop methodology for A/B testing auctions. The accuracy of our method is directly comparable to ideal A/B testing where there is no interference between A and B.
"Price of Anarchy from Data" Abstract: Analysis of welfare in auctions comes traditionally via one of two approaches: precise but fragile inference of the exact details of a setting from data or robust but coarse theoretical price of anarchy bounds that hold in any setting. As markets get more and more dynamic and bidders become more and more sophisticated, the weaknesses of each approach are magnified.
In this paper, we provide tools for analyzing and estimating the empirical price of anarchy of an auction. The empirical price of anarchy is the worst case efficiency loss of any auction that could have produced the data, relative to the optimal.
Our techniques are based on inferring simple properties of auctions: primarily the expected revenue and the expected payments and allocation probabilities from possible bids. These quantities alone allow us to empirically estimate the revenue covering parameter of an auction which allows us to re-purpose the theoretical machinery of Hartline et al. [2014] for empirical purposes. Moreover, we show that under general conditions the revenue covering parameter estimated from the data approaches the true parameter with the error decreasing at the rate proportional to the square root of the number of auctions and at most polynomially in the number of agents. While we focus on the setting of position auctions, and particularly the generalized second price auction, our techniques are applicable far more generally.
Finally, we apply our techniques to a selection of advertising auctions on Microsoft's Bing and find empirical results that are a significant improvement over the theoretical worst-case bounds.