Zhilu Wang and Qi Zhu Win Best Paper Award at the 2022 Design, Automation, and Test in Europe Conference
The team was recognized for its recent paper on certifying robustness of deep neural networks
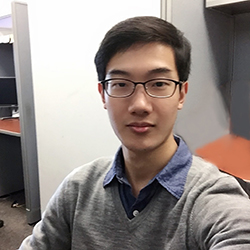
The winning paper titled “Efficient Global Robustness Certification of Neural Networks via Interleaving Twin-Network Encoding,” is coauthored by Chao Huang, lecturer of computer science at the University of Liverpool. Huang is a collaborator in Zhu’s Design Automation of Intelligent Systems Lab.
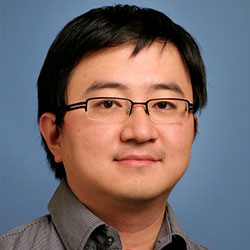
According to Wang, although deep neural networks (DNNs) demonstrate great promise in a wide range of applications, their uncertain behaviors under input disturbances from random noises or malicious attacks are a major hurdle for their adoption in practice, especially for safety-critical systems such as autonomous vehicles and robots.
Building on their prior research in safety-assured design, verification, and adaptation of learning-enabled intelligent systems, the team developed the first global robustness over-approximation method that certifies the robustness of DNNs among the entire input domain with sound and deterministic guarantees.
The method greatly improves efficiency and scalability and can certify DNNs with more than 5,000 neurons, while previous approaches could certify them with up to 64 neurons.
“We will continue improving the efficiency and accuracy of our approach to address larger neural networks and more types of network structures,” Zhu said. “Ultimately, our goal is to enable safe, robust, and secure application of machine learning techniques in practical intelligent systems.”