Career Advice from Sony AI America's Executive Director
Peter Stone spoke with MSAI students about his career path and lessons they could learn from his professional experiences.
Peter Stone was unsure what path he wanted to take after completing his undergraduate degree at the University of Chicago in 1993. He considered going to graduate school to pursue his interest in computer science. He also applied to the Peace Corps and Teach for America.
"I had no idea what to do," Stone said.
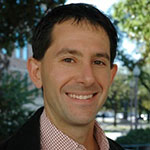
Nearly 30 years later, Stone and Hammond reunited — albeit virtually — as part of the Frameworks in Artificial Intelligence speaker series organized by Northwestern Engineering's Master of Science in Artificial Intelligence (MSAI) program. Hammond, the program’s director, spoke with Stone about his professional journey and what advice he had for current MSAI students.
Here are five tips Stone shared that are applicable to any professional, not just those interested in artificial intelligence.
Know what motivates you
Stone has always been motivated by what he described as challenge problems. How do you make X do Y? He competed in the DARPA Urban Challenge in 2007, when competitors were tasked with building an autonomous vehicle capable of driving in traffic, performing complex maneuvers like parking and passing, and navigating intersections. He took part in RoboCup, where entrants build robots that play soccer against one another. Those were both projects that fit nicely under the same research question that Stone has spent his career pursuing: How can we create complete intelligent agents to interact in dynamic, real-world domains in the presence of teammates and adversaries?
Surround yourself with talented people
In 2015, Stone and two colleagues co-founded Cogitai, a company focused on the machine learning training method known as reinforcement learning. The three made it their mission to hire the most talented people in that area to join the company, and they quickly built a team of roughly 20 of the brightest reinforcement learning PhDs in the country. "Our biggest strength was the people," Stone said.
Recognize when it's time to pivot
In the early days of Cogitai, Stone and his co-founders had big aspirations — moonshot aspirations, as he described them. Within a few years, it became clear the moonshot wasn't going to happen, so the company pivoted.
Build and manage your network
Cogitai was able to pivot because it received funding from Sony. Sony learned about Cogitai because Stone talked about it with Hiroaki Kitano, an AI leader and senior executive at Sony, whom he'd first met at a RoboCup competition. Kitano knew Sony would be interested in Cogitai's mission. That initial conversation led to Stone's current role leading Sony AI America.
Use your advantages
At Sony, Stone leads a team of more than 20 who are focused specifically on reinforcement learning for video games. "The mission of Sony AI is to unleash human imagination and creativity with AI," he said. "We're always thinking about how AI can enhance people's lives." His team recently collaborated with Polyphony, the creative studio behind the Gran Turismo games, and Sony Interactive Entertainment to develop AI technology to race against and elevate the gaming experience for top Gran Turismo drivers.
In addition to his work at Sony, Stone is a full-time professor at the University of Texas, where he is the director of the Learning Agents Research Group. There are benefits to each setting, but Stone explained the clear differences between working in academia and working in industry. "If you're going into industry, you want to work on a problem that (can be productized) in the near term," he said. "In academia, we want to work on problems that are not productizable soon, because otherwise we're just going to get swamped by the greater resources of the bigger tech companies."