Faculty DirectoryAravindan Vijayaraghavan
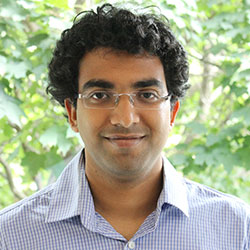
Associate Professor of Computer Science and (by courtesy) Industrial Engineering & Management Sciences
Contact
2233 Tech DriveMudd Room 3011
Evanston, IL 60208-3109
847-467-6145Email Aravindan Vijayaraghavan
Website
Aravindan Vijayaraghavan Homepage
Departments
Education
Ph.D Computer Science, Princeton University
M.A. Computer Science, Princeton University
B. Tech. Computer Science and Engineering, Indian Institute of Technology, Madras
Research Interests
His research interests are broadly in the field of Theoretical Computer Science, particularly, in designing efficient algorithms for problems in machine learning, high-dimensional data analysis, and quantum information. He is also interested in using paradigms that go Beyond Worst-Case Analysis to obtain good algorithmic guarantees.
Selected Publications
- Theoretical Analysis of Weak-to-Strong Generalization NeurIPS 2024. (With Hunter Lang, David Sontag).
- Efficient Certificates of Anti-Concentration Beyond Gaussians FOCS 2024. (With Ainesh Bakshi, Pravesh Kothari, Goutham Rajendran and Madhur Tulsiani).
- New tools for smoothed analysis: least singular values for random matrices with dependent entries STOC 2024. (With Aditya Bhaskara, Eric Evert and Vaidehi Srinivas).
- Error tolerant e-Discovery protocols CSLAW 2024. (With Jinshuo Dong, Jason Hartline and Liren Shan).
- Higher-order Cheeger Inequality for Partitioning with Buffers SODA 2024. (With Konstantin Makarychev, Yury Makarychev and Liren Shan).
- Computing linear sections of varieties: quantum entanglement, tensor decompositions and beyond FOCS 2023. (With Nathaniel Johnston and Benjamin Lovitz).
- Training subset selection for Weak Supervision NeurIPS 2022. (Hunter Lang, Aravindan Vijayaraghavan and David Sontag.)
- A Complete Hierarchy of Linear Systems for Certifying Quantum Entanglement of Subspaces QIP 2023, Physical Review A (journal). (With Nathaniel Johnston and Benjamin Lovitz).
- Agnostic Learning of General ReLU Activation Using Gradient Descent ICLR 2023. (With Pranjal Awasthi and Alex Tang).
- The Burer-Monteiro SDP method can fail even above the Barvinok-Pataki bound. NeurIPS 2022. (With Liam O'Carroll and Vaidehi Srinivas).
- Classification Protocols with Minimal Disclosure. CSLAW 2022. (With Jinshuo Dong and Jason Hartline).
- Efficient Algorithms for Learning Depth-2 Neural Networks with General ReLU Activations. NeurIPS 2021. (With Pranjal Awasthi and Alex Tang).
- Adversarially Robust Low Dimensional Representations. COLT 2021.
- (With Pranjal Awasthi, Vaggos Chatziafratis and Xue Chen).
- Graph cuts always find a global optimum for Potts models (with a catch) ICML 2021.
- (With Hunter Lang and David Sontag).
- Learning a mixture of two subspaces over finite fields. ALT 2021.
- (With Aidao Chen and Anindya De).
- Beyond Perturbation Stability: LP Recovery Guarantees for MAP Inference on Noisy Stable Instances AISTATS 2021. (With Hunter Lang, Aravind Reddy and David Sontag).
- Efficient Tensor Decomposition
- Book chapter in Beyond the Worst Case in the Analysis of Algorithms
- Edited by Tim Roughgarden, Cambridge University Press 2020.
- Adversarial robustness via robust low rank representations.
- (With Pranjal Awasthi, Himanshu Jain and Ankit Singh Rawat). NeurIPS 2020.
- Estimating Principal Components under Adversarial Perturbations.
- (With Pranjal Awasthi and Xue Chen). COLT 2020.
- On Robustness to Adversarial Examples and Polynomial Optimization.
- (With Pranjal Awasthi and Abhratanu Dutta). NeurIPS 2019.
- Smoothed Analysis in Unsupervised Learning via Decoupling
- (With Aditya Bhaskara, Aidao Chen and Aidan Perreault). FOCS 2019.
- Block Stability for MAP Inference
- (With Hunter Lang and David Sontag). AISTATS 2019.
- Towards Learning Sparsely Used Dictionaries with Arbitrary Supports
- (With Pranjal Awasthi). FOCS 2018.
- Clustering Semi-Random Mixtures of Gaussians
- (With Pranjal Awasthi). ICML 2018.
- Optimality of Approximate Inference Algorithms on Stable Instances
- (With Hunter Lang and David Sontag). AISTATS 2018.
- On Learning Mixtures of Well-Separated Gaussians . FOCS 2017. (With Oded Regev).
- Clustering Stable Instances of Euclidean k-means. NIPS 2017. (With Abhratanu Dutta and Alex Wang).
- Approximation Algorithms for Label Cover and the Log-Density Threshold. SODA 2017. (with Eden Chlamtac, Pasin Manurangsi and Dana Moshkovitz).
- Learning Communities in the Presence of Errors. COLT 2016.
- (With Konstantin Makarychev and Yury Makarychev).
- Constant Factor Approximations for Balanced Cut in the random PIE model.STOC 2014.
- (With Konstantin Makarychev and Yury Makarychev). [ Abstract]
- Smoothed Analysis of Tensor Decompositions. STOC 2014.
- (With Aditya Bhaskara, Moses Charikar and Ankur Moitra).
- Bilu-Linial Stable Instances of Max Cut and Minimum Multiway Cut. SODA 2014.
- (With Konstantin Makarychev and Yury Makarychev).
- Approximation algorithms for Semi-random Graph Partitioning problems. STOC 2012.
- (With Konstantin Makarychev and Yury Makarychev).
- Approximating the matrix p-norm. SODA 2011.
- (With Aditya Bhaskara)
- Detecting High Log-Densities -- an O(n1/4) Approximation for Densest k-Subgraph. STOC 2010.
- (With Aditya Bhaskara, Moses Charikar, Eden Chlamtac and Uri Feige).
- On Robustness to Adversarial Examples and Polynomial Optimization.
- (With Pranjal Awasthi and Abhratanu Dutta).
- NeurIPS 2019.[Arxiv|Slides | Abstract]
- Smoothed Analysis in Unsupervised Learning via Decoupling
- (With Aditya Bhaskara, Aidao Chen and Aidan Perreault).
- To appear in FOCS 2019. [ArXiv| Abstract]
- Block Stability for MAP inference
- (With Hunter Lang and David Sontag).
- AISTATS 2019. [ArXiv| Abstract]