Events
CEH Seminar Series
Nurse Workload Balancing Using Real-Time Location Data
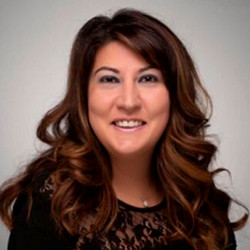
Dr. Beste Kucukyazici, Associate Professor and E. Marie Shantz Fellow of Management Analytics, Smith School of Business, Queen's University
Host: Professor Sanjay Mehrotra
April 8th, 2024
Watch the Seminar Recording Here
Abstract: Sustained inequities in the workload distribution can lead to increased stress, reduced job satisfaction, high turnover and shortages in the nursing team. These imply that healthcare quality could also eventually suffer from the imbalances in nursing workload. We develop a data-driven analytical framework to achieve balanced nurse workloads by optimizing the nurse-patient assignment decisions at the beginning of every shift. To this end, we utilize an extensive data set collected by a real-time location system (RTLS) installed in the surgical services department of a tertiary teaching hospital in Montreal. This enabled us to track the care providers as well as the surgical patients through their journey from the emergency department to the operating room, and the surgical ward. The nurse workload is modeled as a multi-attribute, multilinear function, where the significance of each attribute (for the nurse manager) is elicited using an inverse optimization procedure integrated into a clustering method. This involves inverse optimization with a nonlinear mixed integer forward problem, which has not been studied in the literature. The nurse workload balancing problem is then formulated for the upcoming shift, whereby the nurse-patient assignment decisions constitute the primary lever. This requires deploying the proposed dynamic panel-data model to predict each patient’s required direct care. We also robustify the model to incorporate the uncertainties in the attribute weights. Our findings through the real-life case-study are rather encouraging: The mean variation in total direct care is reduced by 46%. Furthermore, the mean of maximum travel distance and the number of assigned patients are shortened by 65% and 31%, respectively.
Bio: Beste Kucukyazici is an Associate Professor and E. Marie Shantz Fellow of Management Analytics at the Smith School of Business, Queen’s University. She leads Smith's Healthcare Analytics Initiative. Prior to joining Queen's University, Dr. Kucukyazici served as a faculty member at Michigan State University and McGill University. She also held positions at MIT-Zaragoza Logistics Center, St. Mary's Hospital Research Center, and MIT Center for Transportation and Logistics.
Her current research agenda focuses on the development and application of business analytics methods for tackling challenges faced by decision-makers in the health sector and sustainable supply chains. In the context of healthcare management, she specifically studies policy design, healthcare operations management, and medical decision- making. In the context of sustainable operations, she focuses on supply chain network design, analysis of regulations as well as pricing while taking into account the environmental impact and sustainability of the producers’ operations. Dr. Kucukyazici has taught undergraduate and graduate courses on business analytics, operations management, information technology, strategy, healthcare management, and healthcare analytics.
Effects of Geopolitical Strain on Global Pharmaceutical Supply Chain Design and Drug Shortages
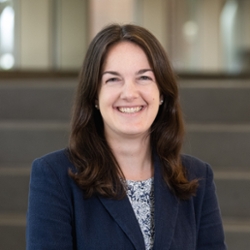
Dr. Emily Tucker, Assistant Professor in the Department of Industrial Engineering and Faculty Scholar in the School of Health, Clemson University
Host: Professor Sanjay Mehrotra
March 11th, 2024
Watch the Seminar Recording Here
Abstract: Pharmaceutical supply chains have experienced on-going struggles to meet the domestic and global demand for drugs for decades. Shortages are caused by supply-demand mismatches and supply strain, including disruptions due to quality and natural disasters. A recent strain – export bans – has emerged as a result of recent geopolitical instability and persistent shortages. These may be mitigated by strategic alliances, but effects on design decisions are unknown. In this work, we present one of the first global supply chain design models for the pharmaceutical industry. We present a stochastic integer programming approach to optimize a company’s supply chain under geopolitical strain, endogenous pricing, strategic alliances, and global demand. The model is solved by integrating Sample Average Approximation and L-shaped methods. We observe large disparities in access by country income level and analyze policies to improve global access.
Bio: Dr. Emily Tucker is an Assistant Professor at Clemson University in the Department of Industrial Engineering and a Faculty Scholar in the School of Health Research. Her research focuses on improving access to social good in systems under strain. She received her PhD and MSE in Industrial and Operations Engineering from the University of Michigan. She was supported by an NSF Graduate Research Fellowship and her dissertation work was awarded the University of Michigan Richard and Eleanor Towner Prize for Outstanding Ph.D. Research. Prior to graduate school, she worked as a Research Health Economist as RTI International and received her BS in Industrial Engineering from NC State.
Optimizing treatment for patients with Localized Renal Masses
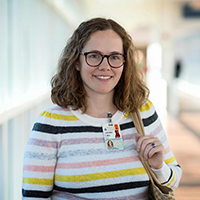
Dr. Jennifer Lobo, Associate Professor in the Department of Public Health Sciences and (by courtesy) Systems and Information Engineering, University of Virginia
Host: Professor Sanjay Mehrotra
November 16th, 2023
Watch the Seminar Recording Here
Abstract: Kidney cancer, or renal cell carcinoma (RCC), is one of the 10 most common cancers in the US. In 2023, over 81,000 people will be diagnosed with RCC. Localized renal masses (LRM, tumors confined to the kidney) make up two-thirds of all RCC. Patients found to have a LRM face many choices on what to do, especially since it is not uncommon for these LRM to be benign. There are currently four ways to manage LRM – active surveillance, ablation, partial nephrectomy, and radical nephrectomy. Current recommendations are based on “expert opinion” and it is typically left up to the doctor to decide what is best for the patient in terms of risk to the kidney and whether the cancer threatens the life of the patient over other medical conditions the patient may have. Dr. Lobo will present a Markov decision process (MDP) model framework for optimizing treatment decision making for patients with LRM. She will present preliminary results, as well as challenges and opportunities of working with electronic medical record data to calibrate models.
Bio: Jennifer Lobo is an Associate Professor in the Department of Public Health Sciences in the School of Medicine at the University of Virginia (UVA), with a courtesy appointment in the Department of Systems and Information Engineering at UVA. She received her B.Sc. in Mathematics from the University of South Carolina, her M.Sc. in Operations Research from North Carolina State University (NCSU), and her Ph.D. in Industrial Engineering from NCSU. Her research primarily involves using Markov models, Markov decision processes, and simulation to inform screening, treatment, and policy decisions for patients with chronic conditions, including kidney cancer, type 2 diabetes, and multiple sclerosis. Her work is currently supported by the National Institutes of Health and the Department of Defense, with additional previous support from the Agency for Healthcare Research and Quality, the National Science Foundation, and the American Cancer Society. Dr. Lobo is an engaged member of INFORMS, currently serving as a Council Member of the Health Applications Society (HAS) and working with the HAS student liaisons. In addition, she serves as an Associate Editor for Health Care Management Science and Area Editor for Mathematical Modelling for Health Systems.
Multi-Criteria Optimization to Inform Colleges’ Academic Operations Response to COVID-19
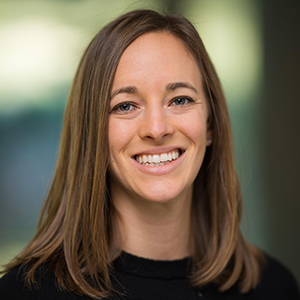
Dr. Lauren Steimle, Assistant Professor in the H. Milton Stewart School of Industrial and Systems Engineering, Georgia Institute of Technology
Host: Professor Sanjay Mehrotra
June 12th, 2023
Watch the Seminar Recording Here
Abstract: Although physical (or “social”) distancing was an important public health intervention during the COVID-19 pandemic, physical distancing dramatically reduced the effective capacity of classrooms. This presented a unique problem to campus planners who hoped to deliver a meaningful amount of in-person instruction in a way that respected physical distancing. This process involved (1) assigning a mode to each offered class as remote, residential (in-person), or hybrid and (2) reassigning classrooms under severely reduced capacities to the non-remote classes. These decisions needed to be made quickly and under several constraints and competing priorities, such as restrictions on changes to the timetable of classes, trade-offs between classroom density and educational benefits of in-person versus online instruction, and administrative preferences for course modes and classrooms reassignments. We solve a flexible integer program and use hierarchical optimization to handle the multiple criteria according to priorities. We show that our optimization model is able to provide a significant improvement on several metrics representing the amount of physically-distanced in-person instruction delivered when compared to a strategy in which no rooms are reassigned. We discuss how this model informed an iterative and collaborative decision-making process with the Georgia Tech COVID-19 Task Force throughout the summer of 2020.
Bio: Lauren Steimle is an Assistant Professor in the H. Milton School of Industrial and Systems Engineering at the Georgia Institute of Technology. Her research interests include optimization, stochastic modeling, and predictive modeling with applications in public health and medical decision-making. She received her Bachelor’s degree in Systems Science and Engineering from Washington University in St. Louis and her Ph.D. in Industrial and Operations Engineering from the University of Michigan. She is a recipient of the IISE Transactions Best Paper Award, INFORMS Service Science Best Cluster Paper Award Finalist, the NSF Graduate Research Fellowship, and 3rd place in the New England Journal of Medicine SPRINT Data Analysis Challenge. Her work has been supported by the Centers for Disease Control and Prevention and a graduate fellowship from the National Science Foundation.
Data Analytic Models and Insights in Adaptive Treatment of Chronic Depression
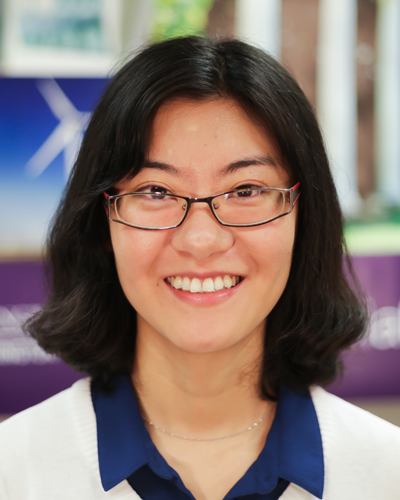
Dr. Shan Liu, Associate Professor in Industrial and Systems Engineering and Adjunct Associate Professor in Radiology, University of Washington
Host: Professor Sanjay Mehrotra
Monday, May 8th, 2023
Watch the Seminar Recording Here
Abstract: Evidence-based healthcare modeling research is increasingly in demand due to excessive costs and the desire to improve quality of care in the U.S. Our goal is to identify optimal strategies to improve population health outcomes under limited resources, while accounting for patients’ heterogeneity in disease progression and population dynamics. This talk will feature research at the UW Healthcare Analytics Lab using advanced analytics to tackle one urgent problem facing Americans today—depression. Mitigating depression has become a national health priority as it affects 1 out of 10 adults in the U.S. We design predictive and optimization models aiming to adaptively monitor and treat chronically depressed patients. Prognostic-based monitoring that stratifies patients’ disease risk and adaptively allocates treatment resources to high-risk patients may improve overall health outcomes. Our objective is to translate data into solutions regarding who should be monitored and treated at the appropriate time, and study how cost-effective these adaptive strategies could be.
Bio: Shan Liu is an associate professor of Industrial & Systems Engineering at the University of Washington. She received her Ph.D. in Management Science & Engineering from Stanford University, a S.M. in Technology and Policy from MIT, and a B.S. in Electrical Engineering from The University of Texas at Austin. Her research focuses on the design and evaluation of healthcare interventions to improve patients’ health and enable cost-effective care delivery. She develops methods in sequential decision making, decision analytics, and systems modeling to optimize healthcare systems in areas such as hepatitis C, mental health, influenza, COVID-19, trauma, and HIV. She has received multiple grants from the National Science Foundation and National Institutes of Health. She is an associate editor for Healthcare Management Science, and currently serving as secretary to the INFORMS Health Applications Society.
Personalizing Tuberculosis Treatment Adherence Support
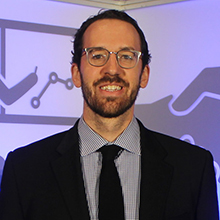
Dr. Justin J. Boutilier, Charles Ringrose Assistant Professor in Industrial and Systems Engineering and Faculty Affiliate in Emergency Medicine, University of Wisconsin-Madison
Host: Professor Sanjay Mehrotra
Monday, April 10th, 2023
Watch the Seminar Recording Here
Abstract: Tuberculosis (TB) is a global health priority and ending the TB pandemic is part of the United Nations Sustainable Development Goals. Lack of patient adherence to treatment protocols is the main barrier to reducing the global disease burden of tuberculosis. In this talk, we will study the operational design of a treatment adherence support platform that requires patients to verify their treatment adherence on a daily basis. To do this, we partner with Keheala, a TB treatment adherence support provider in Kenya and use data from a completed randomized controlled trial. First, we investigate who should be enrolled on the platform by evaluating who benefits from treatment adherence support. We use a causal forest framework to estimate heterogenous treatment effects and demonstrate that differentiated care can improve program efficiency and reduce inequity in treatment outcomes. Second, we use an empirical framework to quantify the impact of human “support sponsor” outreach on subsequent patient verification behavior. Finally, we develop an algorithm that approximates one step of policy iteration and relies on machine learning to personalize support sponsor outreach. Our analysis establishes that patient verification can be increased by personal sponsor outreach and that patient behavior data can be used to identify at-risk patients for targeted outreach. This work represents an important step towards shifting the current TB treatment adherence paradigm from observational (i.e., monitoring and collecting data on patient adherence) to actionable (i.e., combining behavioral data with analytics to improve patient adherence in a personalized manner).
Bio: Justin J. Boutilier is the Charles Ringrose Assistant Professor in Industrial and Systems Engineering and a Faculty Affiliate in Emergency Medicine at the University of Wisconsin – Madison. His research focuses on combining optimization and machine learning to improve the quality, access, and delivery of healthcare in a variety of settings. He is particularly interested in global health projects involving emergency response or digital health technologies, and he has ongoing projects with local stakeholder’s in India, Kenya, and Colombia. Justin’s impactful work has been recognized by INFORMS: his papers have once been runner-up (2020) and twice finalist (2021, 2022) for the Pierskalla Best Paper Award, honorable mention for the Doing Good with Good OR Paper competition (2021), and as a PhD student, Justin won the Seth Bonder Scholarship for Applied Operations Research in Health Services (2017). Justin received his B.Sc. in Mathematics and Statistics from Acadia University, and his Ph.D. in Operations Research from the University of Toronto. Prior to joining the University of Wisconsin, he was a postdoctoral associate with the Humanitarian Supply Chain Lab and the Center for Transportation and Logistics at MIT.
Learning Policies for Allocating Scarce Housing Resources to People Experiencing Homelessness
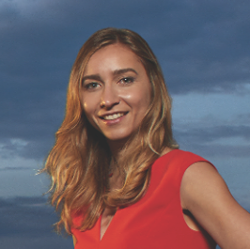
Dr. Phebe Vayanos, WiSE Gabilan Assistant Professor and Assistant Professor of Industrial and Systems Engineering and Computer Science, USC
Host: Professor Sanjay Mehrotra
This seminar has been postponed
Abstract: We study the problem of allocating scarce housing resources of different types to individuals experiencing homelessness based on their observed covariates. We leverage administrative data collected in deployment to design an online policy that maximizes mean outcomes while satisfying budget and fairness requirements. We propose a policy in which an individual receives the resource maximizing the difference between their mean treatment outcomes and the resource bid price, or roughly the opportunity cost of using a resource. Our approach has nice asymptotic guarantees and is easily interpretable. We show results on real data from the Homeless Management Information System in LA: our policies improve rates of exit from homelessness by 1.2% and policies that are fair in either allocation or outcomes by race come at very low price of fairness. In addition, to help guide the discussion among stakeholders in deciding on appropriate fairness requirements to impose when allocating scarce resources, we propose a framework for evaluating fairness in such resource allocation systems and present a set of incompatibility results that investigate the interplay between them.
Bio: Phebe Vayanos is a WiSE Gabilan Assistant Professor of Industrial & Systems Engineering and Computer Science at the University of Southern California. She is also an Associate Director of CAIS, the Center for Artificial Intelligence in Society at USC. Her research is focused on Operations Research and Artificial Intelligence and in particular on optimization and machine learning. Her work is motivated by problems that are important for social good, such as those arising in public housing allocation, public health, and biodiversity conservation. Prior to joining USC, she was lecturer in the Operations Research and Statistics Group at the MIT Sloan School of Management, and a postdoctoral research associate in the Operations Research Center at MIT. She holds a PhD degree in Operations Research and an MEng degree in Electrical & Electronic Engineering, both from Imperial College London. She has served as a member of the ad hoc INFORMS AI Strategy Advisory Committee and as VP of Communications for the INFORMS Section on Public Sector Operations Research. She is an elected member of the Committee on Stochastic Programming (COSP) and an Associate Editor for Operations Research Letters and Computational Management Science. She is a recipient of the NSF CAREER award and the INFORMS Diversity, Equity, and Inclusion Ambassador Program Award.
Modeling and Optimization for Population Health Screening Policies
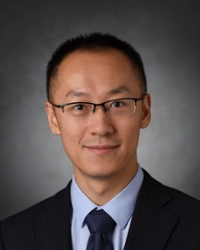
Dr. Qiushi Chen, Assistant Professor of Industrial and Manufacturing Engineering, Penn State University
Host: Professor Sanjay Mehrotra
February 13th, 2023
Watch the Seminar Recording Here
Abstract: Population health screening can be an effective approach for identifying diseases at early stages to enable timely treatment and to improve long-term health outcomes. The rationale is simple, but how to develop efficient screening policies at the population level while considering limited resources is nontrivial. This talk will discuss several studies we have conducted in modeling and optimizing screening policies in a variety of clinical contexts across infectious diseases, chronic diseases, and developmental disabilities. We will discuss the policy insights for screening—either common or unique to these clinical contexts—that are drawn from our modeling analyses and future research opportunities in the emerging era of big healthcare data.
Bio: Qiushi Chen is an Assistant Professor in the Harold and Inge Marcus Department of Industrial and Manufacturing Engineering at the Pennsylvania State University. He earned his PhD degree in Operations Research from the Georgia Institute of Technology in 2016 and completed his post-doctoral training at Massachusetts General Hospital and Harvard Medical School. His research has focused on utilizing innovative mathematical modeling, optimization, and data analytics tools, integrated with real-world healthcare datasets, to better inform clinical decisions and policy makings in broad healthcare settings. He has been collaborating closely with researchers from medicine, health policy, health economics, and social sciences in multidisciplinary research projects on modeling for chronic diseases, behavioral and mental health, and opioid and substance use. His work has been supported by research grants from the National Institutes of Health. He is currently serving on the Council of INFORMS Health Applications Society.
Gaming the Heart Allocation System to Jump the Queue
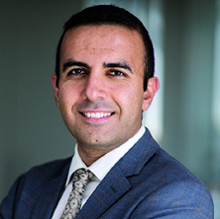
Dr. Sait Tunc, Assistant Professor of Industrial and Systems Engineering, Virginia Tech
Host: Professor Sanjay Mehrotra
November 14th, 2022
Watch the Seminar Recording Here
Abstract: Heart transplantation remains the standard treatment option for patients with advanced heart failure. However, the success of this life-saving therapy is limited by the scarcity of donor hearts. Whereas organ scarcity is a pervasive problem for all major organ transplant systems, the US heart transplantation system has the following important distinctive feature: Transplant candidates are prioritized according to the severity of their pre-transplant medical therapy – the more severe the therapy, the higher the priority. The underlying premise for this rule is that the severity of a patient’s therapy accurately reflects her medical urgency for receiving a heart transplant. However, it is widely suggested that this rule opens up room to game the system. Although the existence of this gaming phenomenon is widely recognized, the specific conditions that produce this behavior are not well understood; missing in the literature is a systematic analysis of this problem. This talk will focus on addressing this shortcoming. We will discuss our novel model studying the gaming decisions of transplant centers and the underlying trade-offs, and try to address the questions: Under what conditions does gaming occur, i.e., do transplant center doctors have an incentive to assign high-severity therapies to low-urgency patients, and to what extent? And how can it be prevented within the confines of the current system?
Bio: Sait Tunc is an Assistant Professor of Industrial and Systems Engineering at Virginia Tech. He earned his Ph.D. in industrial engineering from the University of Wisconsin-Madison in 2017. He then spent two years as a postdoctoral researcher at the University of Chicago Booth School of Business before joining Virginia Tech in 2019. Tunc's research focuses on application-driven theoretical problems and utilizes large-scale real-world databases, with particular emphasis on medical decision making and operational efficiency in health care delivery systems. Some of his recent research has focused on operational problems in organ transplantation including developing risk prediction and intervention frameworks to identify and save organs at high risk of discard, designing patient- and center-level nudge mechanisms to decrease organ wastage, studying the problem of systematic gaming in heart transplantation, and analyzing the strategic decisions of transplant centers under competition. He is also interested in identifying disparities in COVID-19 spread-, hospitalization- and mortality-rates as well as to determine the geographical and racial inequalities in the outcomes, and using these in determining the optimal allocation and delivery of mitigation resources.
An Optimization-based Scheduling Framework for Multi-service Appointment Systems with Non-stationary Arrival Processes
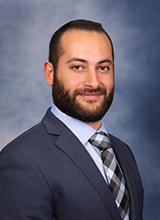
Dr. Hrayer Aprahamian, Assistant Professor of Industrial and Systems Engineering, Texas A&M University
Host: Professor Sanjay Mehrotra
September 12th, 2022
Watch the Seminar Recording Here
Abstract: In this work, we analyze multi-service appointment systems with non-stationary arrival processes. The main goal is to identify server schedules that lead to good performing systems, which we measure through the expected waiting time and number of customer rejections. This decision problem arises in a number of applications and is especially relevant when certain service types dominate others. A key challenge in this analysis is the lack of closed-form analytical expressions that characterize the performance of the system. In this work, we construct a stylized optimization model that approximates the original stochastic system. An analysis of a problem instance comprised of a single service type leads to key structural properties which we use to devise a globally convergent solution scheme that runs in polynomial time. This solution scheme is then generalized to the case of multiple service types for two different formulations of the problem. To demonstrate the effectiveness of the proposed framework, we conduct a case study on Texas A&M University’s College and Psychological Services. Our results demonstrate that our optimal solutions substantially improve the performance of the system over current practices and increase access to critical mental health services by as much as 55%.
Bio: Dr. Hrayer Aprahamian is an Assistant Professor at the Wm. Michael Barnes Department of Industrial and Systems Engineering at Texas A&M University. He received his Ph.D. in Industrial and Systems Engineering from Virginia Tech in 2018, and was co-advised by Drs. Ebru K. Bish and Douglas R. Bish. Dr. Aprahamian’s research interests lie at the interplay between combinatorial/discrete and continuous optimization, with particular interest in applications related to healthcare systems and public policy decision-making. His research papers have appeared in journals such as Management Science, INFORMS Journal on Computing, Stochastic Systems, and IISE Transactions. He was a finalist for the 2021 JFIG Paper competition, received the IISE Transactions Award in 2020, the Pritsker Doctoral Dissertation Award in 2019, the Paul E. Torgersen Research Excellence Award in 2018, and was runner-up for the 2017 Pierskalla Best Paper Award.
Estimating the Prevalence of Multiple Chronic Diseases via Maximum Entropy
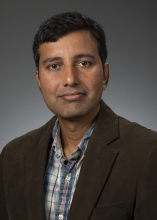
Dr. Hari Balasubramanian, Associate Professor of Industrial Engineering, University of Massachusetts, Amherst
Host: Professor Sanjay Mehrotra
August 8th, 2022
Watch the Seminar Recording Here
Abstract: Patients with multiple chronic conditions, also known as multi-morbidity in the clinical literature, have a disproportionate impact on the U.S. healthcare system. According to a 2017 report by RAND, 19% of Americans (around 60 million individuals) had four or more chronic conditions, and they account for more than 50% of total healthcare expenditure. A baseline predictive model for the probabilities of co-occurring conditions is essential for quantifying epidemiological associations between condition groups, resource planning for targeted interventions, and driving decision support for personalized medicine. However, MCC patients exhibit significant heterogeneity in chronic condition combinations, and the number of individuals in a disease dataset is usually small compared to the number of possible disease combinations. Therefore, simple maximum-likelihood estimates of disease co-occurrence will erroneously assign zero probabilities to disease combinations that are missing from the dataset but are likely to occur in the larger population. In this work, we combine maximum-entropy optimization, data mining, and machine learning techniques to create an algorithm, called MaxEnt-MCC, for estimating the prevalence of chronic diseases in a population in the face of sparse data. In a case study using Medical Expenditure Panel Survey (MEPS) data, we show how MaxEnt-MCC can be used to predict previously unobserved but likely disease combinations, quantify associations between groups of chronic conditions, and estimate healthcare costs in a principled manner.
This research is joint work with Peter Haas (Professor, College of Information and Computer Sciences, University of Massachusetts, Amherst) and Pracheta Amarnath (PhD student, College of Information and Computer Sciences, University of Massachusetts, Amherst).
Bio: Dr. Hari Balasubramanian is Associate Professor of Industrial Engineering at the University of Massachusetts, Amherst. He received his doctoral degree at Arizona State University in 2006. After graduation, Dr. Balasubramanian spent two years as a Research Associate at Mayo Clinic in Rochester, Minnesota before joining the University of Massachusetts in 2008. His research interests are in operations research applied to healthcare. Specific applications have included capacity planning and scheduling in outpatient, inpatient and emergency room settings. Dr. Balasubramanian's work has been supported by grants from the National Science Foundation including a National Science Foundation CAREER award (2013-2019) focused on improving primary care delivery. His papers have been published in both operations research as well as clinical journals. His recent work is on modeling the impact of care interventions on patients with complex medical and social needs.
2022 IPHAM Population Health Forum & 10th Anniversary
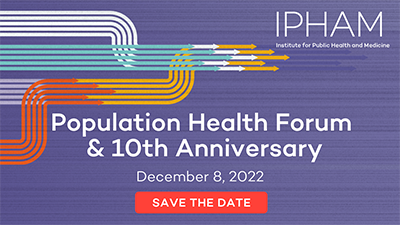
The 2022 IPHAM Population Health Forum & 10 Year Anniversary Celebration will be held on Thursday, December 8, 2022 from 9 AM - 1:30 PM. The event will feature:
- Welcome and 10-year review with IPHAM Director, Ronald T. Ackermann, MD, MPH
- Keynote lecture by John A. Rich, MD, MPH, inaugural Director of RUSH BMO Institute for Health Equity
- Poster Session
- Awards Ceremony
HEALTH APPLICATIONS SOCIETY ONLINE SEMINAR SERIES:
This seminar series welcomes a broad range of healthcare modeling research topics such as healthcare operations, medical decision making, health policy, and health analytics. The series is organized by Sanjay Mehrotra (Northwestern University), Sait Tunc (Virginia Tech), Qiushi Chen (Pennsylvania State University).
The advisory board of the Year 2022 includes Mark Van Oyen (University of Michigan), Maria Mayorga (North Carolina State University), and Timothy Chan (University of Toronto).
Seminars are typically held from 12PM - 1PM CT on the fourth Friday of every month. More information on the series, as well as links to past seminars, can be found here.
Some Thoughts on Causal Inference with Observational Data
HAS Speaker Dr. Stefan Scholtes
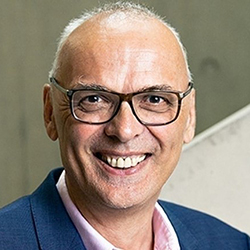
Dr. Stefan Scholtes, University of Cambridge
Friday, September 23rd
Abstract: Most papers in empirical healthcare operations are based on observational data. The limitations in terms of making causal inferences are well known and researchers will typically resort to one of a handful of “identification strategies” popularised in the econometrics literature. These methods are based on untestable assumptions and referees will often question their validity. Editors then end up having to make a judgement call whether or not there is reason to believe that the assumptions are valid beyond reasonable doubt. That’s an unsatisfactory state of affairs. Is there an alternative to this “single model” evidence production? I will argue that it can be more informative if researchers present a well-documented journey through different model specifications, including some traditional causal models. This allows them to shed light on the data from different angles and enables readers to form a more robust judgement of the validity of the estimated effect directions and sizes. I will use a recent paper to exemplify this approach.
Bio: Stefan Scholtes is Dennis Gillings Professor of Health Management and Professor of Operations and Technology Management at Judge Business School, University of Cambridge. His research and teaching focuses on health system design and management and on service innovation, and is strongly practice-based and embedded in close collaborations with executives and clinicians in health systems, hospitals and primary care practices in the UK and the USA. He is founding director of the Cambridge Centre for Health Leadership & Enterprise, chair of the board of the largest primary care practice in the East of England, and co-founder of the East of England Joint Evidence and Intelligence Cell established in 2020 between the NHS and Cambridge Judge Business School to support COVID planning. He holds honorary appointments with Cambridge University Hospitals and the Royal Papworth Hospital in Cambridge, and a courtesy appointment with the Cambridge University Engineering Department. Stefan is founding Department Editor of the Healthcare Management Department of Management Science and has held editorial positions with Operations Research and Mathematics of Operations Research.
Leveraging Population-Based Cohorts for Pandemic Response
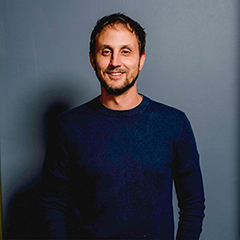
Dr. Rafael Araos, Universidad del Desarrollo, Santiago, Chile
Friday, August 26th
Abstract: During the Covid-19 pandemic, countries needed to develop SARS-CoV-2 surveillance platforms rapidly and leverage already existing administrative datasets to design and execute their responses. Thus, timely access to representative data for decision-making and evaluating interventions emerged as a critical need for managing current and future health threats. Using real-time frequentist and Bayesian approaches for data analysis and modeling and leveraging an electronic, country-wide, prospective cohort, we developed tools for allocating testing resources, guiding isolation measures, and estimating hospitalization needs and vaccine effectiveness. Providing the authorities with scientific evidence to support their decisions was crucial for increasing public trust and engagement. Based on our and others’ experience, we foresee that electronic, administrative cohorts will become invaluable public health tools. To integrate these efforts and maximize funding opportunities, stakeholders should be aware of the available platforms.
Bio: Dr. Rafael Araos is a physician scientist trained in Internal Medicine and Infectious Diseases. He is currently an associate professor of medicine at Universidad del Desarrollo in Santiago, Chile, and an active member of the Chilean National Pandemic Response Committee, the Covid-19 advisory group with the Chilean Ministry of Health (MoH). During the first 2 years of the Covid-19 pandemic, he served as Chief Epidemiologist at the Chile MoH and Covid-19 advisor. During that time, his group implemented a national scale surveillance system integrating multiple administrative datasets that allow the country to generate real time data on Covid-19 vaccine effectiveness and other health outcomes. His team also implemented public repositories of data for others to analyze the pandemic dynamics and impact and to educate the public in collaboration with academia. Their work has been extensively published and has helped to shape vaccination policies globally, highlighting the impact that large-scale research platforms can have if they are timely deployed and supported. For the last ten years, Dr. Araos has received funding from the US Centers for Disease Control and Prevention (CDC) and more recently from the China CDC as well, to develop platforms for assessing the burden and impact of antimicrobial resistance and improving surveillance of respiratory viruses and vaccine effectiveness.
IPHAM SEMINAR SERIES:
Lifestyle Medicine for Mental Health: Towards Health Equity
Alyssa Vela
October 20th, 2022
This presentation will discuss the intersection between mental health and lifestyle medicine and how to leverage a lifestyle medicine approach to support mental health and psychological wellbeing. The presentation will also discuss how the emerging field of lifestyle medicine can support a shift towards mental health equity.
Guest:
Alyssa Vela, PhD, DipACLM
Assistant Professor of Surgery (Cardiac Surgery) and Psychiatry and Behavioral Sciences
Northwestern University Feinberg School of Medicine
Dr. Alyssa Vela is a clinical health psychologist and board-certified Lifestyle Medicine provider. She works with the Cardiac Behavioral Medicine team at Northwestern's Bluhm Cardiovascular Institute and is the co-chair of the Department of Surgery Diversity, Equity, and Inclusion Committee.
Design and Implementation of a Surgical Decision Aid with Predictive Analytics
Patricia Franklin
November 3rd, 2022
Osteoarthritis affects almost two-thirds of aging adults. When knee or hip osteoarthritis pain limits daily activities, patients and clinicians must decide if joint arthroplasty surgery is indicated. This PCORI-funded research designed a patient-clinician decision report that includes predicted surgical outcomes based on national data. The report was implemented and evaluated among 6000 patients at 12 sites. Qualitative and quantitative evaluations of the decision report's impact on care will be presented.
Guest:
Patricia D. Franklin, MD, MBA, MPH
Professor, Departments of Medical Social Sciences, Medicine (Rheumatology), Orthopaedics
Northwestern University Feinberg School of Medicine
Patricia Franklin, MD MBA MPH advances learning healthcare systems through designing and implementing novel methods to translate knowledge to improve patient care and population health outcomes. Her recent research designs and evaluates methods to collect, analyze, and report patient-reported and clinical data to guide personalized treatment decisions among aging adults with knee and hip osteoarthritis. Dr. Franklin has held diverse leadership roles including medical director of health system quality, co-director of training programs for junior faculty and post-doctoral trainees, and principal investigator of large federally funded research studies.
Past Events
CEH Seminar Series
Prioritizing Hepatitis C Treatment Decisions in U.S. Prisons
Turgay Ayer, an assistant professor at Georgia Tech, spoke at the CEH Seminar Series on Wednesday, October 5, 2016.
Learn more
CEH Seminar Series
Online Decision-Making with High-Dimensional Covariates
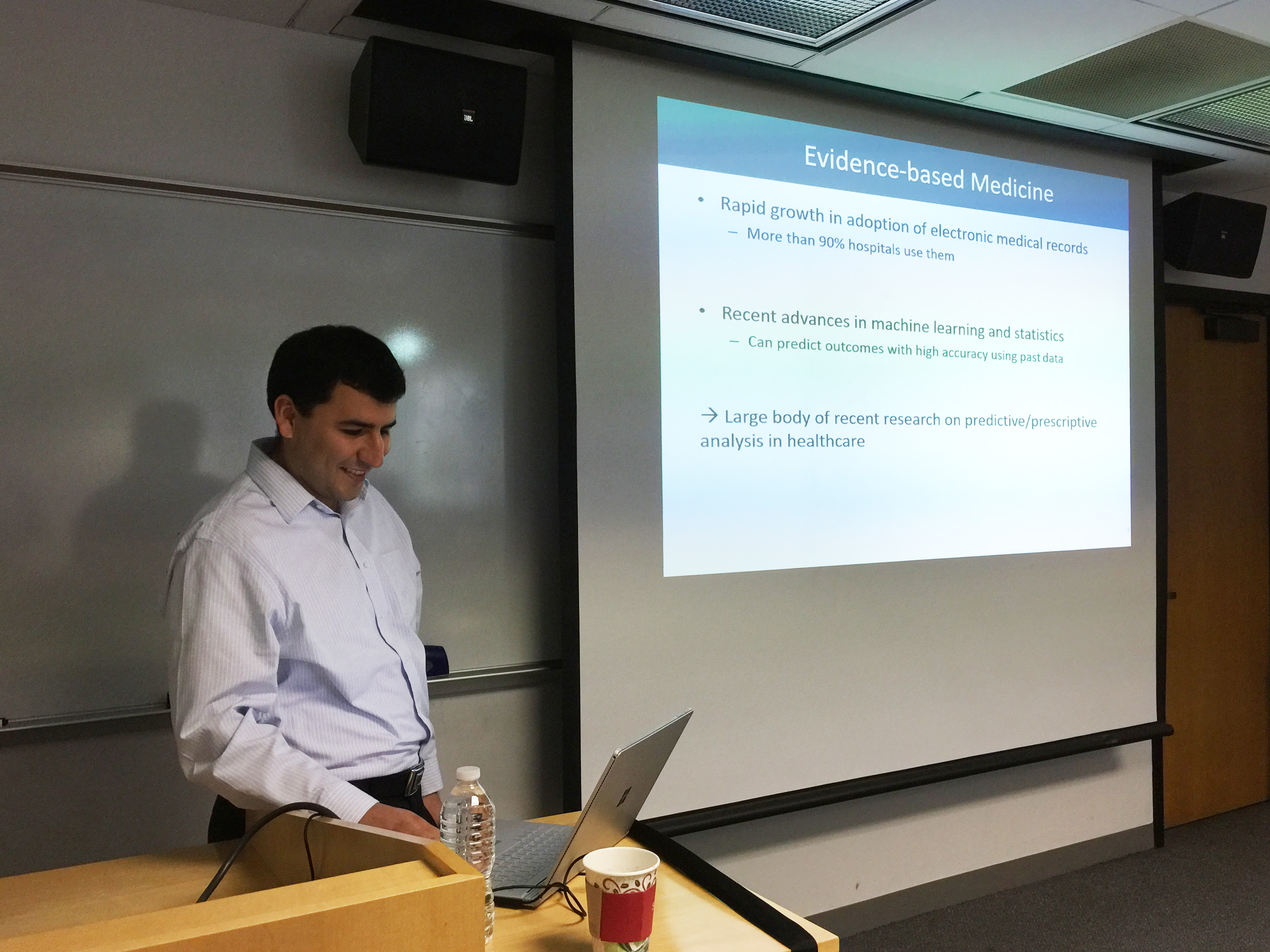
Mohsen Bayati, an associate professor at Stanford University, spoke at the CEH Seminar Series on Wednesday, September 28, 2016.
Learn more