News, Blog, Events
UPCOMING EVENTS:
Spring Seminar Speaker: To Be Announced
PAST EVENTS:
The Balance Between Model Performance and Interpretability in Demand Forecasting
Jeff Tackes
Sr. Manager of Data Science, Kraft Heinz
February 1st, 2024
In the rapidly evolving landscape of demand forecasting, businesses increasingly push the forecast accuracy limits by looking to new “State of the Art” models to predict market trends and consumer behavior. However, the complexity of these models often comes at the cost of interpretability, posing significant challenges for data scientists and decision-makers. This talk aims to address the critical balance between leveraging the predictive power of complex, 'black box' models and maintaining the interpretability necessary for strategic business applications. Our discussion will begin by exploring the latest advancements in time series forecasting and discussing their strengths and limitations. We will delve into the intricacies of models that, while powerful, often function as black boxes, making it difficult to understand the 'why' behind their predictions. This opacity can hinder trust and adoption in business environments where understanding the reasoning behind forecasts is as crucial as the forecasts themselves.
To bridge this gap, we introduce SHAP (SHapley Additive exPlanations) values, a cutting-edge approach in model interpretability. SHAP values, grounded in cooperative game theory, provide a robust framework to decipher the contribution of each feature to the prediction of a complex model.
The talk will include real-world examples of how many businesses today decide on how to deploy machine learning into their business practices.
Speaker Bio:
Jeff Tackes is Sr. Manager of Data Science for Kraft Heinz, headquartered in Chicago, IL. With over 10 years of industry experience, Jeff has developed a deep understanding of the intricacies of demand forecasting and has successfully built best-in-class demand forecasting systems for leading fortune 500 companies.
Jeff is known for his data-driven approach and innovative strategies that optimize forecasting models and improve business outcomes. He has led cross-functional teams in designing and implementing demand forecasting systems that have resulted in significant improvements in forecast accuracy, inventory optimization, and customer satisfaction. Jeff's expertise in statistical modeling, machine learning, and advanced analytics has enabled him to develop cutting-edge forecasting methodologies that have consistently outperformed industry standards. Jeff's strategic vision and ability to align demand forecasting with business goals have made him a trusted advisor to senior executives and a sought-after expert in the field.
Recent Investigations in Machine Learning and Edge Computing
Rajeev Shorey
Former CEO of the University of Queensland - IIT Delhi Academy of Research (UQIDAR), Adjunct Faculty in Computer Science & Engineering, IIT Delhi
November 20th, 2023
In this talk, we highlight a few research challenges in the intersection of Machine Learning and Edge Computing. More specifically, we look at the Federated Learning paradigm in a faulty edge ecosystem. In this talk, we first analyze the impact of the number of edge devices on an FL model and provide a strategy to select an optimal number of devices that would contribute to the model. We observe the impact of data distribution on the number of optimal devices. We then investigate how the edge ecosystem behaves when the selected devices fail and provide a mitigation strategy to ensure a robust Federated Learning technique. Finally, we design a real-world application to highlight the impact of the designed mitigation strategy. The talk will end with a brief discussion of several open research problems in the intersection of Machine Learning and Edge Computing.
Speaker Bio:
Dr. Rajeev Shorey is the former CEO of the University of Queensland – IIT Delhi Academy of Research (UQIDAR) at IIT Delhi, India. Rajeev serves as an adjunct faculty in the Computer Science & Engineering department at IIT Delhi. Rajeev received his Ph.D. and M.S. (Engg) in Electrical Communication Engineering from the Indian Institute of Science (IISc), Bangalore, India in 1996 and 1991 respectively. He received his B.E degree in Computer Science and Engineering from IISc, Bangalore in 1987. Rajeev’s career spans several reputed research labs – TCS Research & Innovation, General Motors (GM) India Science Laboratory, IBM India Research Laboratory and SASKEN Technologies. Rajeev’s work has resulted in more than 75 publications in international journals and conferences and several US patents, all in the area of wireless and wired networks. He has 13 issued US patents and several pending US patents to his credit. For his contributions in the area of Communication Networks, Rajeev was elected a Fellow of the Indian National Academy of Engineering in 2007. He was recognized by ACM as a Distinguished Scientist in December 2014. He was elected a Fellow of the Institution of Engineering and Technology (IET), UK in 2021. He was selected as the Distinguished Lecturer of the IEEE Future Networks Technical Community in October 2023.
The Role of Simulation in Digital Transformation: A Focus on Digital Twin Solutions
Peyman Davoudabadi
Senior Director of Global Strategic Initiatives, ANSYS, Inc.
October 25th, 2023
Digitalization has the potential to create trillions of dollars in socioeconomic value, according to the World Economic Forum. In the digital transformation of products lifecycle, engineering simulation plays a critical role in evaluating multiple designs and operating scenarios before building prototypes. With the emergence of Artificial Intelligence (AI) and the Internet of Things (IoT), a connected simulation-based model of a product or process, known as a digital twin, has become possible. Sensors connected to the physical product or process provide real-time data to the digital twin, which in turn offers additional data and insights. These insights help determine the root cause of performance problems, evaluate various performance optimization and maintenance strategies, and improve companies’ bottom line by perfecting the next generation of products and processes.
In this presentation, Dr. Peyman Davoudabadi will discuss the role of simulation in digital transformation, with a focus on use cases that demonstrate how integrating simulation with AI and IoT solutions can enable companies to quickly analyze current or past operating conditions, rapidly diagnose issues, predict future behavior, and optimize product performance.
Speaker Bio:
Dr. Peyman Davoudabadi is the Senior Director of Global Strategic Initiatives at ANSYS, Inc. He is globally responsible for the strategy, operation, technical direction, and successful delivery of Industry Verticalization, Platform APIs, Cloud, HPC, and Customer Success Management.
Prior to his current role, Dr. Davoudabadi led the North America Ansys Customer Excellence team across Ansys' New and Emerging Technologies, including Simulation Process and Data Management, Material Lifecycle Management, Process Integration Design Optimization, Model-Based System Engineering, Digital Twins, Embedded Software, and Functional Safety. During his tenure at Ansys, Dr. Davoudabadi has architected multiple innovative processes and platform solutions, driving significant business outcomes and integrating technologies to deliver enterprise-level solutions that minimize time to value. He has also established and led engineering teams focused on industry, applications, and strategic business development, and has led complex, multidisciplinary projects across various industry verticals. Dr. Davoudabadi earned his MBA from Northwestern Kellogg in 2019 and his PhD in Mechanical Engineering from the University of Illinois at Chicago in 2008, where his research focused on modeling and simulation of dusty plasma systems.
Enabling Smart Innovation at Caterpillar with Analytics
Dan Reaume, Chief Analytics & AI Director, Caterpillar Inc.
April 12th, 2023
By applying analytics to the data streaming from its vast fleet of connected assets, Caterpillar provides an enhanced experience for its customers and dealers. This talk outlines the IoT infrastructure underlying these capabilities and highlight the opportunities they enable. It then explores the analytics techniques applied to transform raw sensor data into actionable insights. Finally, the talk concludes with a discussion of future possibilities enabled by recent breakthroughs in AI.
Speaker Bio:
Dan Reaume has over 25 years of experience leading the development and delivery of analytic solutions and strategies for several of the world’s largest and most iconic companies. After completing his Ph.D. in Industrial and Operations Engineering at the University of Michigan, Dan joined General Motors R&D, where he was awarded GM’s top technical award on three occasions, rose to the rank of technical fellow, and led GM’s Senior Leadership Technical Council. He subsequently held leadership positions with Dow Corning, Dow Chemical, and Revenue Analytics before assuming his current role leading Caterpillar’s Analytics and Artificial Intelligence Center of Excellence. The Center provides thought leadership to Caterpillar and applies cutting-edge techniques in areas such as advanced diagnostics, AI-enhanced condition monitoring, and electrification. Dan has a long track record of service to the Analytics profession, teaching at the University of Michigan’s College of Engineering for almost two decades, serving on the INFORMS Roundtable, and serving on advisory boards for Purdue, Michigan State and Wayne State Universities, the Chicago Analytics Leadership Network, and the Chicago CDO Community. Dan is also a licensed professional engineer and attorney.
NVIDIA Omniverse: AI and Reinforcement Learning Applications
Kelly Guo
Wednesday, February 1st, 2023
Nvidia Omniverse is a platform supporting the creation and development of 3D worlds and AI tools. It accelerates not only complex 3D workflows, but also enables ground-breaking new ways to visualize, simulate, and code the next frontier of ideas and innovation. In this talk, we will look at AI applications and frameworks within Omniverse that encompass a wide range of domains, including computer vision, animation, and robotics. Leveraging the power of end-to-end GPU-accelerated reinforcement learning pipelines, Omniverse Isaac Sim provides a reinforcement learning framework that combines high-fidelity physics simulation with high-speed performance. We will look at examples from quadruped locomotion to complex dexterous manipulation tasks, as well as explore sim-to-real transfer of bringing policies trained purely in simulation to physical robots running in the real world.
Speaker Bio
Kelly Guo is a senior applied research engineer at NVIDIA, where she works on projects at the intersection of machine learning and simulation technology. She's an active developer on the Isaac Gym team, with a focus on reinforcement learning frameworks and environments. She also spends a lot of her time in Omniverse, working on projects around robotics, animation, and pose estimation. More recently, Kelly's been leading development efforts for integrating Isaac Gym and reinforcement learning features into Isaac Sim, where we hope to combine fast reinforcement learning with other amazing features in Isaac Sim and Omniverse.
MLOps for Deep Learning
ODSC West 2022
San Francisco, CA
Yegna Subramanian and Professor Diego Klabjan will be presenting their work on the center's DELOS project at ODSC West 2022.
Abstract:
In model serving, two important decisions are when to retrain the model and how to efficiently retrain it. Having one fixed model during the entire often life-long inference process is usually detrimental to model performance, as data distribution evolves over time, resulting in a lack of reliability of the model trained on historical data. It is important to detect drift and retrain the model in time. We present an ensemble drift detection technique utilizing three different signals to capture data and concept drifts. In a practical scenario, ground truth labels of samples are received after a lag in time, which we consider appropriate. Our framework automatically decides what data to use to retrain based on the signals. It also triggers a warning indicating a likelihood of drift.
Model training in serving is not a one-time task but an incremental learning process. We address two challenges of life-long retraining: catastrophic forgetting and efficient retraining. To solve these two issues, we design a retraining model that can select important samples and important weights utilizing multi-armed bandits. To further address forgetting, we propose a new regularization term focusing on synapse and neuron importance.
Only a significant minority of companies unlock the true potential of AI as trained models accumulate dust due to challenges in MLOps. Serving reliable AI predictions to customers involves cost, effort, and planning to set up a continuous deployment pipeline. MLOps for Deep Learning demands a carefully crafted deployment pipeline. We discuss our open-source project which is a robust continuous deployment pipeline by integrating our unique drift detection and model retrain algorithms for serving DL models. We show how to efficiently deploy, monitor, and maintain DL models in production using our solution which is a Kubernetes native POC solution.
MLOps World: Machine Learning in Production Conference
June 10th, 2022
Toronto, Canada
MLOps Summit San Francisco
June 16th, 2022
San Francisco, California
Digital & Virtual Health Care: Current state and emerging trends
Opportunities to apply data science & Artificial Intelligence
PLAMEN PETROV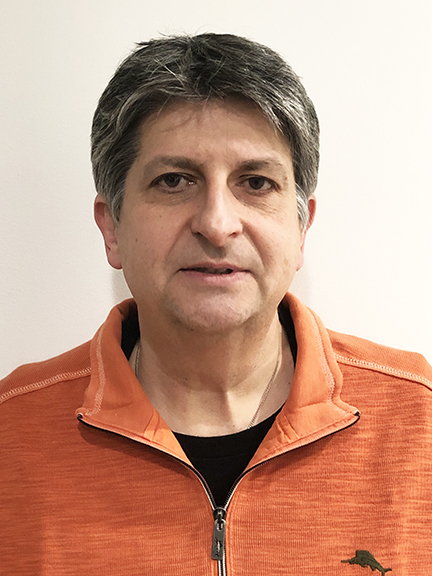
Thursday, April 14th, 2022
The global telehealth market size was $144 billion in 2020. The telehealth market is projected to grow to $636 billion in 2028 at a CAGR of 32.1% in the forecast period, 2021-2028. Data science and Artificial Intelligence have proven to be critical components of virtual health solutions allowing clinicians to make real-time, data driven medical decisions at the point of care and to scale the telehealth services to millions of patients, achieving improved health outcomes and superior patient experience. In this talk, will discuss the current state and emerging trends of the virtual health industry with a focus on the applicability of machine learning and data science to this rapidly growing field.
Speaker Bio
Plamen Petrov is Chief Technology Officer at Hydrogen Health. Hydrogen Health is an AI-powered digital health company established by Anthem Inc, Blackstone Growth and K Health. Hydrogen Health’s mission is to partner with employers to close the primary care gap through access to comprehensive whole-health care. Hydrogen’s virtual primary care solution brings K Health’s suite of digital healthcare services to employers to address prevention and chronic condition management while also providing world-class support for acute care needs. With 5 million direct-to-consumer users and 2 million insured members, the unique technology is powered by millions of medical records and data sets, in partnership with the Mayo Clinic Platform, Maccabi Healthcare Services, and Anthem.
Prior to Hydrogen Health, Plamen was VP of Data Science and Chief Data Officer at Anthem Inc. Plamen has extensive experience in industry and academia having worked in different roles at companies such as Anthem, KPMG, IBM, Deloitte LLP, Blue Cross Blue Shield Association, United Airlines, Sun Microsystems, Motorola, and Cincinnati Bell. Plamen is a recognized expert applying Artificial Intelligence technologies and data science methods to various business domains and in particular on probabilistic reasoning and inference, Natural Language Processing (NLP) and Machine Learning. Plamen holds a Master of Engineering Management degree and Ph.D. in Computer Science. Plamen serves on the faculty of the University of Illinois at Chicago where he conducts research, advises students, and teaches graduate courses.
Accelerating Deep Learning with Dask and GPUs
STEPHANIE KIRMER
Tuesday, APRIL 27
Deep learning has and continues to produce astonishing opportunities and advancements in machine learning, but compute availability and speed can create barriers to further progress. For data scientists, moving from single machine to cluster computing infrastructure opens up a world of new possibility - projects, datasets, and models that were previously too large or complex to use can be within reach when GPU clusters are involved.
In this talk, I will discuss how performing deep learning tasks on Dask GPU clusters is more accessible and more affordable than one might think. I will describe Dask briefly, talk about the importance of GPUs, and demonstrate some of the surprising acceleration made possible by taking a training job from single node to a Dask GPU cluster.
Speaker Bio
Stephanie Kirmer is a Senior Data Scientist at Saturn Cloud, a company making large scale Python easy and accessible to the data community using Dask. She has applied machine learning and data science in a number of industries, including as a data science technical lead at a travel data startup, and as a senior data scientist at Uptake, an industrial data science company. Before joining Uptake, she worked on data science for social policy research at the University of Chicago and taught sociology and health policy at DePaul University. To learn more, visit www.stephaniekirmer.com.
Watch the recording here.
Analytic Applications to Change the World
Timothy Chou 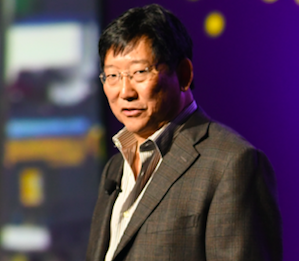
Tuesday, November 10, 3 p.m. - 4 p.m. CST
This talk is organized as three mini-TED talks. In the first I will talk about a new class of software: enterprise analytic/AI applications. For too long the state of the art of analytics and data science has been based on the "wrong rock" method. It's time to take the next steps that we've already seen happen in enterprise workflow applications. Next, while there is a lot of data generated by People, it is dwarfed by what is generated by Things. Unfortunately, most of the techniques for the Internet of People don't work for the Internet of Things. I will discuss five big challenges in connecting and collecting data from Things. Finally, I would like to challenge the audience to use their talents to truly change the world. Twenty-five percent of the globe's population will be in Africa in less than twenty years. All developing economies share the needs for the basic infrastructure of power, water, food, education, and healthcare. Will we try and repeat what we did in the first world, or do we have an opportunity to re-invent the future? As a small example I will close with a discussion of our Pediatric Cloud Project - a project to connect all 1,000,000 healthcare machines in all the children's hospitals in the world and through software and data transform children's healthcare.
Speaker Bio
Timothy Chou began his career at one of the original Kleiner Perkins startups, Tandem Computers. He has had a long career in enterprise software. He served as President of Oracle On Demand beginning in 2000, which was the beginning of Oracle's multi-billion dollar cloud business. Today he serves on the board of directors of two public companies: Blackbaud and Teradata. In parallel to his commercial career he started teaching introductory computer architecture at Stanford University in 1982, and after leaving Oracle he returned to Stanford and launched the first class on cloud computing.
Listen to Timothy on The Not Mini Adults Podcast, where he discusses the power of the Cloud and what it could mean for children’s healthcare and research.
Diego Klabjan Featured on the Data Mindset Podcast
Diego Klabjan was interviewed for the Data Mindset Podcast. He discusses contemporary topics in deep learning, and about bringing up the next generation of students in the arena.
REFIT: aRtificial intElligence For Internet of Things
Matthew Alvarez MSCS ’21, Aditya Sinha MSIT ‘20, Nancy Zhang MSiA ’20, J Montgomery Maxwell MS ESAM ‘20
CDL Blog Post 9/3/2020
In recent years the popularity of the Internet of Things (IoT) ecosystem has exploded. One area of interest in the IoT landscape is the combination of IoT infrastructure and Machine Learning. Traditionally, such systems use existing streaming solutions (e.g. AWS Kinesis, Azure Stream Analytics, Google Cloud Platform Stream Analytics), however, existing solutions typically require a significant amount of work to integrate and support a specific use case.
Northwestern University’s Center for Deep Learning is developing an open-source streaming platform that is designed to seamlessly integrate IoT workloads with modern machine learning approaches. The aRtificial intElligence For Internet of Things (REFIT) system is designed to be highly scalable and fault tolerant by using production-tested open source technologies. REFIT is also developed using Helm, a package manager for Kubernetes. Using these technologies enables you to deploy REFIT as a helm chart using a cloud provider (such as AWS, GCP, or Azure), on premises, or locally using minikube.
Note: local deployments on minikube are only recommended for development or testing
The primary goals of REFIT are as follows:
- Produce predictions for a stream of events
- Continually improve the predictive component of the system
- Scale to meet elastic demand of IoT infrastructure
Some secondary goals include:
- Secure data in transit & at rest
- Integrate with upstream components
- Multitenancy
System Configuration
REFIT is meant to be a highly configurable system that can support many IoT use-cases out of the box. To get REFIT to work for your use case, you only need to provide a “project schema” for your project. Your project schema is a YAML file that consists of a few pieces of key information and a set of fields that can come from your IoT infrastructure.
System Design
REFIT’s implements a publish-subscribe architecture, using Apache Pulsar to communicate between individual components in the system. In addition to Pulsar, REFIT uses Apache Cassandra to persist data on disk and Redis as an in-memory cache to store Machine Learning models that are ready for use. In addition to these components, REFIT uses four services to ingest, train, predict, and visualize data from upstream IoT components.
Ingestion Service: The REFIT Ingestion Service is an Apache Camel application that is designed to integrate with a variety of upstream systems and services. The Ingestion service’s purpose is to receive sensor data from existing IoT infrastructure, translate the information to an internal protocol buffer representation, and to send the serialized data to the Inference Service.
AI Application: The AI Application component of the REFIT system is where the machine learning models are created. Periodically the AI application will read historical data from the database and create a new machine learning model. After creating a new model that is ready for use, the AI application will save the model to an in-memory data store and notify the inference service of the new model.
Inference Service: The Inference Service is an Apache Flink streaming application that consumes events from the IoT infrastructure and events from the AI Application. Using Flink’s Stateful Function API the Inference Service is able to extract features from IoT data and create predictions using the machine learning model provided by the AI Application. After the Inference Service extracts features and creates a prediction, it will publish an event for the Integration Service to consume.
Integration Service: The Integration service is the final internal service in the REFIT application. Much like the Ingestion Service, the Integration Service is an Apache Camel application that is designed to integrate with a variety of downstream systems and services. The Integration Service receives event data along with predictions from the Inference Service, encrypts the data and routes data to REFIT’s Cassandra database. The Integration service also implements a Grafana Implementation that allows you to visualize event data and predictions in real time. In addition to the Integration Service’s existing functionality, you can configure the application to integrate with many upstream systems using Camel’s many components or by creating your own custom component.
Grafana: REFIT’s integration service enables the system to easily integrate with many other applications. One such application is Grafana, which REFIT uses to allow you to visualize and monitor data streams in real time. REFIT’s modular approach allows the system to dynamically generate dashboard templates that can act as a base to visualize, manipulate, and learn from the trends in the data.
Efficient Architecture Search for Continual Learning
July 16, 2020
Diego Klabjan, Qiang Gao, and Zhipeng Luo have submitted a new paper for publication that addresses a novel approach to achieve higher classification accuracy in continual learning with neural networks. Read about CLEAS.
New A.I. Tool Created by Center for Deep Learning Team Featured in Northwestern News
June 18, 2020
CAVIDOTS provides easy-to-skim summaries of academic papers. Read the full article here, and a detailed blog post below.
Center for Deep Learning creates COVID-19 query tool, organizing key information for medical researchers in a large multi-document dataset
Ning Wang, Diego Klabjan, Han Liu
CAVIDOTS — Corona Virus Document Text Summarization
April 22, 2020
The coronavirus has severely endangered public health and damaged economies. To provide medical researchers with more efficient tools to acquire relevant and also salient information to fight the virus, we developed a query tool, CAVIDOTS (CoronA VIrus DOcument Text Summarization) accessible here for investigating COVID-19 related academic articles. (Full Article can be found HERE)
Inaugural Center for Deep Learning Symposium Looks to Future of Artificial Intelligence
November 14, 2019
At its inaugural symposium on November 14, Northwestern Engineering’s Center for Deep Learning, a community of deep learning-focused data scientists who conduct research and collaborate with industry, convened members of academia along with current and potential corporate partners to discuss the state of the technology and the best path forward. Full Article can be found HERE
Center for Deep Learning Presents: Machine Learning on Google Cloud
Yufeng Guo
Developer, Advocate, Machine Learning, Google
Thursday, August 22, 2019
This interactive session will focus on the tools for doing machine learning Google Cloud Platform (GCP). From exploration, to training, to model serving, we will talk about available tools and how to piece them together, depending on your needs. We will explore some common patterns, as well as take time to address any questions, so bring use cases with you to discuss.
BIO: Yufeng is a Developer Advocate at Google focusing on Cloud AI, where he is working to make machine learning more understandable and usable for all. He is the creator of the YouTube series AI Adventures, at yt.be/AIAdventures, exploring the art, science, and tools of machine learning. He enjoys hearing about new and interesting applications of machine learning, so share your use cases with him on Twitter@YufengG.
Thoughts on autoML
Diego Klabjan Blog Post 7/29/19
Diego Klabjan Follow
Director, Center for Deep Learning; Director, Master of Science in AnalyticsThere are many start-ups screaming Data Science as a Service (DSaaS). The shortage of data scientists is well document and thus DSaaS makes sense. However, data science is complex and steps such as feature engineering and hyper parameter tuning are tough nuts to crack.
There are several feature selection algorithms with varying degree of success and adoption, but feature selection is different from feature creation which often requires domain expertise. Given a set of features, feature selection can be automated, but the latter is still unsolvable without substantial human involvement and interaction.
On the surface hyper parameter tuning and model construction are further down the automation and self-service path thanks to the abundant research work and commercial offerings of autoML. Scholars and industry researchers have developed many algorithms for autoML with two prevailing approaches of Bayesian optimization and reinforcement learning. Training of a new configuration can now be aborted early and perhaps restarted later if other attempts are not as promising as the first indicators were showing. Training of a new configuration has also been improved by exploiting previously obtained weights. In short, given a particular and unique image problem autoML can without human assistance configure a CNN-like network together with all other hyper-parameters (optimization algorithm, mini-batch size, etc). Indeed, autoML is able to construct novel architectures that compete and even outperform hand-crafted architectures and to create brand new activation functions.
Google’s autoML which is part of their Google Cloud Platform have competed in Kaggle’s competitions. On older competitions autoML would have always been in top ten. In a recent competition autoML competed ‘live’ and finished second. This attests that automatic hyper parameter tuning can compete with best humans.
There is one important aspect left out. autoML requires substantial computing resources. On deep learning problems it often requires weeks and even months of computing time on thousands of GPUs. There are not many companies that can afford such expenses on a single AI business problem. Even Fortune 500 companies that we collaborate with are reluctant to go down this path. If organizations with billions of quarterly revenue cannot make a business case for autoML, then it is obvious that scholars in academia cannot conduct research in this area. We can always work on toy problems, but this would take us only so far. The impact would be limited due to unknown scalability of proposed solutions and publishing work on limited computational experiments would be hindered. A recent PhD student of mine recently stated “I do not want to work on an autoML project since we cannot compete with Google due to computational resources.” This says a lot.
The implication is that autoML is going to continue to be further developed only by experts in tech giants who already have in place computational resources. Most if not all of the research will be left out of academia. This does not imply that autoML is doomed since in AI it is easy to argue that research in industry is ahead of academic contributions. However, it does imply that the progress is going to be slower since only one party is going to drive the agenda. On the positive side, Amazon, Google, and Microsoft have a keen interest in improving their autoML solutions as part of their cloud platforms. It can be an important differentiation factor driving customers.
Before autoML becomes more used in industry, the computational requirements must be lowered, and this is possible only with further research. I guess we are at the mercy of FAANG (like we are for many other aspects outside autoML) to make autoML more affordable.
Autonomous Cars: Sporadic Need of Drivers (without a Driver’s License)
Diego Klabjan Blog Post 6/25/19
Diego Klabjan Follow
Director, Center for Deep Learning; Director, Master of Science in Analytics
Based on SAE International’s standard J3016 there are 6 levels of car automation with level 0 as the old fashion car with no automation and the highest, level 5, offering full automation in any geographical region and (weather) condition with a human only entering the destination. As a reference point, Tesla cars are at level 3 (short sellers claim it is level 2 while Elon believes it is level 5 or soon-to-be-level-5).
Waymo is probably the farthest ahead with level 4 cars – full automation on select types of roads and geographical regions (“trained” in Bay Area or Phoenix, but not in both), and weather conditions (let the car not drive in Chicago in snow or experience pot holes when snow is not on the ground). Atmospheric rain storms in San Francisco have probably also wrack havoc to Waymo’s cars.
In “Self-driving cars have a problem: safer human-drive ones,” The Wall Street Journal, June 15, 2019, Waymo’s CEO John Krafcik stated that level 5 was decades away. A human will be needed to occasionally intervene for foreseeable future (a flooded street in San Francisco or a large pot hole in Chicago).
Let us now switch to the other side of the equation: humans, aka drivers. Apparently, they will be needed for decades. The existence of humans in the future is less problematic except for the believers of superintelligence and singularity, but the survival of drivers is less clear.
I have three young-adult children, each with a driver’s license, however they are likely the last generation with driving knowledge. Ten years from now I actually doubt they will still know how to drive. While they currently have their own car at home owned by the parenty, they frequently use car-sharing. I doubt they will ever own their car since they will not need one. Their number of miles driven per year is steadily going down and I am confident that five years from now it is going to deplete to zero. As with any other skill or knowledge, if you do not practice it, you forget how to do it.
Thirty years from now I predict that the only people with driving knowledge will be those who are currently between 30 and 45 years old (I am assuming that all of us older than 45 will not be delighted to drive at the age of 75 or will RIP). Those who are now 30 years old or younger will forget how to drive in a decade or so. Those currently below the driving age and yet-to-be-born will probably never even learn how to drive.
People outside of this age range of between 30 and 45 will not be able to own a car unless we get level 5 cars, which seems to be unlikely. No matter how much they long to have a car, occasionally they will have to take control of the car, but they will not know how to operate it. As a result, there will be no car ownership for those outside of age 30 to 45.
The logic does not quite add up since car-sharing drivers will always be needed to transport my children, and occasionally, me. In short, thirty years from now, the only drivers will be those who are currently between 30 and 45 years old or are, and will be, car-sharing drivers. The latter will be in a similar situation as the airplane pilots are now. Car-sharing drivers will mostly be trained in simulators just to have enough knowledge to sporadically take control of a car. Since we consider today’s pilots to know how to fly, we should as well call future car- sharing operators, drivers.
There is another problem with not having level 5 cars soon. I personally find Tesla’s autopilot useless (I do not own a Tesla, and thus, this claim is based on reported facts), as well as, level 4 cars. The main purpose of an autonomous car is to increase my productivity. The car should drive while I am working on more important things. If I have to pay attention to the road and traffic even without actively driving, it defeats the purpose; it is still a waste of time. The only useful autonomous cars are level 5. There is clearly a benefit of levels 1-4 by being safer, but at least, in my case, this argument goes only so far.
In summary, the future of car automation levels 0-4 is bleak. They do increase safety, but they do not increase productivity if a human driver needs to be in the car paying attention to weather and pot holes. Furthermore, the lack of future drivers will make them even more problematic. In short, a human in the loop is not resounding.
One solution is to have level 4 cars being remotely assisted or teleoperated. This practice is already being advocated by some start-ups (Designed Driver) and, in essence, is a human on the loop. In such a scenario, I will be able to do productive work while being driven by an autonomous car with teleoperated assistance. This business model also aligns nicely with the aforementioned lack of drivers since there will only be a need for ‘drivers’ capable of driving in a simulator. Is this a next generation job that will not be wiped out by AI? You bet it is, and we will probably need many.
There is a challenge on a technical side. A car would have to identify an unusual situation or low-confidence action in order to invoke a teleoperator. If this can be done with sufficient reliability is yet to be seen. Current deep learning models are capable of concluding “this is an animal that I have not yet seen” without specifying what kind of animal it is. There is hope to invent deep learning solutions RELIABLY identifying an unseen situation and passing the control to a teleoperator.
Northwestern Engineering Launches New Center for Deep Learning
NU News - 9/25/18
As artificial intelligence grows in prominence, Northwestern Engineering is launching the Center for Deep Learning , which will build a community of deep learning-focused data scientists to service the research and industry needs of the Midwest.
Led by faculty in Computer Science and Industrial Engineering and Management Sciences, the interdisciplinary center will produce academic research and technological solutions in collaboration with corporate partners, ranging from Fortune 500 corporations to startups and from financial technology to pharmaceuticals.
At the September 24 kickoff, representatives of approximately 30 companies attended the kickoff meeting at the newly renovated Seeley G. Mudd Building.
“It’s a wide range of people from diverse industries,” said Jim Bray, director of Northwestern’s Office of Corporate Engagement. “There’s broad applicability of deep learning to different industries.”
NVIDIA, the Santa Clara, California-based company which created the graphics processing unit (GPU), is the inaugural partner with the center. The company has donated high-performance hardware that the center is already utilizing for deep learning research.
Diego Klabjan , professor of industrial engineering and management sciences and director of the Master of Science in Analytics program, co-directs the initiative with Doug Downey , associate professor of and computer science, and Mark Werwath , director of the Master of Engineering Management program and co-director of the Farley Center for Entrepreneurship and Innovation.
“The center is an opportunity for folks in the industry to apply this new technology and have it actually provide business impact,” Downey said. “It’s also affords us as academics an opportunity to gain more insight into the kinds of problems that arise when you try to take this technology out of the center and have impact as a real-world product.”
Within the first year, Downey said, the center hopes to create technology that results in both academic papers and business value for multiple companies.
“They know that the expertise is coming from places like Northwestern. They come here to hire some of the best data scientists in the world,” Werwath said. “It’s all very synergistic in the sense that it’s all a win-win-win.”
Corporate partners will benefit from the talent moving through the center, able to recruit Northwestern students well versed in deep learning.
“We see a lot of interest from companies in this space, a lot of interest in students coming out in this field, and it’s a hot job market right now,” said Tim Angell, senior associate director at Northwestern Corporate Engagement. “There’s recognition that Northwestern has strength in this area.”
Diego Klabjan Blog Post
5/24/18
Imagine an AI OS allowing the power of AI to be harnessed seamlessly by system builders, without the need for extensive model building or framework-specific engineering.
Imagine an open and accessible forum bringing together industry players, startups and academics collaborating to create real-world solutions.
Imagine democratizing cutting edge AI to transform foundational research, technology innovation, and education by building an open source AI operating system and ecosystem.
At Northwestern University, your imagination has become reality. Our Deep Learning Lab, created this year, understands AI’s rapid rise and importance in industry and society. New applications like self-driving cars, personalized medicine, personalized advertising and marketing, virtual assistants, and more are expected to revolutionize industries in coming years.
Bringing about this future will require new advances in several areas, from fundamental advances in learning and inference techniques, to new paradigms for programming AIs making the technology widely accessible, to infrastructure challenges like reliability, efficiency, and security. Deep Learning Lab will be a global leader in those areas.
The lab envisions collaborative research projects in:
- Bioinformatics
- Image and video AI
- Personalization (marketing, product design, etc)
- Optimization
- Natural language processing (NLP)
- Internet of Things (IoT)
Sponsors of Deep Learning Lab, like NVIDIA, are excited about helping define the future of deep learning and AI technologies. Sponsors also gain access to top Northwestern researchers, student interns, graduates, and more.